Highlighted Research Projects
NRT-AI: AWARE-AI: AWAREness for Sensing Humans Responsibly with AI (AWARE-AI NRT Program)
Cecilia O. Alm, PI
Reynold Bailey, Co-PI
Ferat Sahin, Co-PI
Kristin Shinohara, Co-PI
Esa Rantanen, Co-PI
Senior Personnel or Affiliated Faculty:
S.P. (Rain) Bosworth
Gabriel Diaz
Garreth Tigwell
Matt Huenerfauth
Alex Orobia
Roshan Pieris
Bing Yan
Chris Kanan (UR)
Leslie Weber, NRT Project Coordinator
National Science Foundation
Sensing-based artificial intelligence (AI) systems use information gathered from humans to make predictions and respond to humans in numerous applications. As these systems become more widespread, enormous research challenges are merging. These AI systems must react responsibly and flexibly, respect diversity and privacy, and achieve trustworthiness to avoid unintended consequences at a larger societal scale. To address these challenges, gaps in graduate education programs related to AI will need to be bridged to create a growing and sustainable pipeline of well-rounded AI scientists and engineers who understand software, hardware, human-computer interaction, and human cognitive aspects of this technology, as well as ethical considerations. To ensure AI technology is well-designed to improve all citizens’ productivity, welfare, and safety, it is also vital to build an inclusive research workforce. This National Science Foundation Research Traineeship (NRT) award to the Rochester Institute of Technology will provide unique training to a diverse student body, whose members will be future research leaders in developing responsible, human-aware AI technologies. The project anticipates training 75 master’s and Ph.D. students, including 25 funded trainees, from computing and information sciences, engineering, mathematics, psychology, and imaging science. The Awareness for Sensing Humans Responsibly with AI (AWARE-AI) NRT project will enhance U.S. competitiveness in AI and help develop a diverse workforce by providing funded traineeships to Deaf and Hard of Hearing, female, African American, Latino/a American, and Native American students.
REU Site: Computational Sensing for Human-centered AI
Cecilia O. Alm, PI
Reynold Bailey, Co-PI
Jamison Heard, SP
Kristen Shinohara, SP
Zhi Zheng, SP
Roshan Peiris, SP
Tracy Worrell, Facilitator
Tammi Wickson, Logistics Manager
National Science Foundation
We propose a 3-year REU Site on Computational Sensing for Human-Centered Artificial Intelligence. With the boundaries between HCI and AI blurring, and AI growing increasingly agile and pervasive, the next generation of computational scientists must be capable of responsibly and effectivelyleveraging a spectrum of multisource sensing data from human beings. REU students will gain experience with sensing hardware and software towards transformative advances in intelligent systems. We will address two limitations in Al: first, that underserved populations are at risk of being ignored with the present focus on big data AI and, second, that AI trainees often lack experience with human subjects data collection and the fostering of critical thinking about human-elicited datasets. Enabling diverse stakeholders early in their careers agency in discovering how to collect, fuse, make inferences with and visualize multimodal human data helps nurture a workforce that can transform how humans and machines presently engage and collaborate. For our Site’s next iteration, we set ambitious and attainable goals: (1) to advance fundamental research in multimodal computational sensing for human-centered AI in team-based research
projects that couple human study with sound AI experimentation focused on modestly-sized and inclusive datasets, (2) to develop our programmatic mechanisms for aiding students to transition confidently from being taught to being research mentored by high-profile research faculty, with the aim to ensure students’ readiness for entering computer science PhD programs, and (3) to enhance strategies aimed at doubling the representation of Native American or Latina/o students and students with disabilities among our applicant pool, and reach even more demanding selection targets for exceptionally diverse cohorts.
The National General Aviation Flight Information Database (NGAFID)
Travis Desell, PI
DOT Federal Aviation Administration (FAA)
The purpose of this work is to maintain and further develop the National General Aviation Flight Information Database (NGAFID) and its integration within the Federal Aviation Administration's Aviation Safety Information Analysis and Sharing (ASIAS) System. The NGAFID provides a free to use interface for institutions and private pilots to upload flight data, which can be analyzed to track trends in exceedences (potential flight issues), reanimate nights for educational purposes, and provide more advanced interfaces to determine flight safety at fleet level. A major focus of this work is in using AI and machine learning on geospatial and time series data to determine potential flight hazards and accident precursors to make general aviation safer.
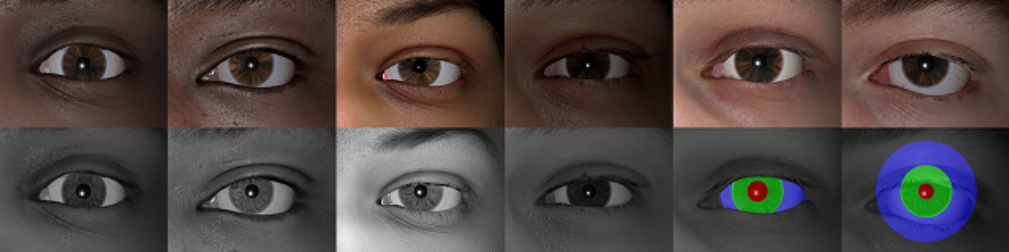
Improved Semantic Segmentation with Natural Gaze Dynamics
Gabriel Diaz, PI
Reynold Bailey, Co-PI
Alex Orobia, Key Personnel
Meta Reality Labs
Current methods for semantic segmentation of eye imagery into pixels that represent the skin, sclera, pupil, and iris cannot leverage information about how the eye moves across time, or gaze dynamics. This limitation is a symptom of the datasets on which models are trained, which reflect individual, temporally non-contiguous frames of the eyes looking at known locations. To address this issue, we will develop a computer graphics rendering pipeline for the generation of datasets that represent sequences of eye movements faithful to natural gaze dynamics. In addition, we will develop models that leverage these dynamics in the semantic segmentation of eye imagery on the next frame through a process of short-term prediction. Finally, these models will be used in a series of studies designed to evaluate the potential benefit of segmentation models that have been trained using synthetic gaze sequences when applied to real-world recordings of gaze sequences.
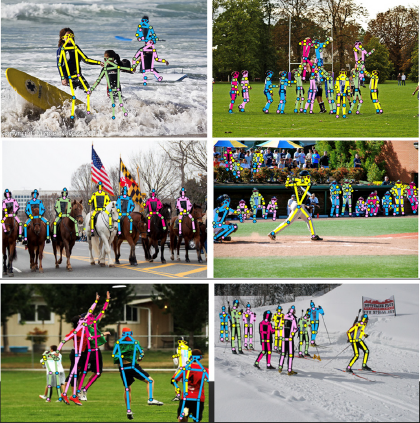
Collaborative Research: Multimethod Investigation of Articulatory and Perceptual Constraints on Natural Language Evolution
Matthew Dye, PI
Andreas Savakis, Co-PI
Matt Heunerfauth, Co-PI
Corrine Occhino, Key Personnel
National Science Foundation
The evolution of language is something that appears to be unique to the human species. Viewed as a cognitive tool, language is a powerful system responsible for the cultural and technological advance of human civilization. Like physical tools, such as the wheel or fire, cognitive tools have the power to shape their user. That is, languages need not only evolve by change within linguistic systems themselves, but also through changes in the organisms (humans) that use those languages. The research proposed here will focus upon one particular type of human linguistic system - signed languages - and on one aspect of human organisms - spatial visual attention. It will ask the fundamental question: to what extent have signed languages evolved over generations to conform to the human visual and articulatory systems, and to what extent is the human visual attention system shaped by the use of a signed language within the lifetime of an individual signer?
Twenty-First Century Captioning Technology, Metrics and Usability
Matt Huenerfauth, PI
DHHS ACL/Gallaudet University
Captioning plays an important role in making video and other media accessible for many people who are Deaf or Hard of Hearing. This collaborative research project with Gallaudet University will investigate the design of metrics that can predict the overall quality of text captions provided during video for people who are Deaf or Hard of Hearing (DHH). In addition, this project will study the presentation, display, and user experience of people who are DHH viewing captions. This project will include focus groups, interviews, surveys, and experimental studies with several thousand DHH participants across the U.S., with studies occurring at Gallaudet, at RIT, and at other U.S. locations, to obtain input and feedback from a diverse cross-section of the U.S. DHH population. Through this work, we will identify key requirements from stakeholders as to the quality of captions, identify factors that can be used to implement software-based automatic metrics for evaluating caption quality, and identify new methods for modifying the presentation and display of captions, to boost the overall user experience of DHH users. This project will create software tools, as well as a captioned video collection, which will serve as a critical research infrastructure for empirical research on caption quality and accessibility. Outcomes of this project include the creation of information materials and outreach to the DHH community about captioning technologies, as well as software and standards for how to best evaluate captions of videos for these users.
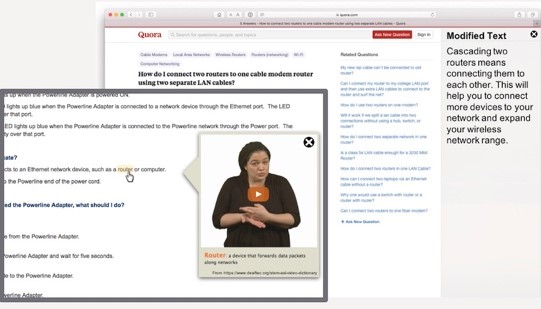
Automatic Text-Simplification and Reading-Assistance to Support Self-Directed Learning by Deaf and Hard-of-Hearing Computing Workers
Matt Huenerfauth, PI
National Science Foundation
While there is a shortage of computing and information technology professionals in the U.S., there is underrepresentation of people who are Deaf and Hard of Hearing (DHH) in such careers. Low English reading literacy among some DHH adults can be a particular barrier to computing professions, where workers must regularly "upskill" to learn about rapidly changing technologies throughout their career, often through heutagogical (self-directed) learning, outside of a formal classroom setting. There has been little prior research on self-directed learners with low literacy nor on automatic text-simplification reading-assistance systems for DHH readers, who have a unique literacy profile. Our interdisciplinary team includes researchers and educators with expertise on DHH computing education, natural language processing researchers with expertise in text simplification technologies, and accessibility researchers with expertise in conducting empirical studies with large numbers of DHH users evaluating assistive technologies. RIT is an ideal setting for this study, as it is home to the National Technical Institute for the Deaf, including DHH computing students who engage in workplace experiences as part of a senior capstone course, in which they must heutatogogically learn about new technologies for work projects. As a research vehicle for this study, we will implement a web-browser plug-in that provides automatic English text simplification (on-demand) for DHH individuals, including providing simpler synonyms or sign-language videos of complex English words or simpler English paraphrases of sentences or entire documents. By embedding this prototype for use by DHH students as they learn about new computing technologies for workplace projects, we will evaluate the efficacy of our new technologies.
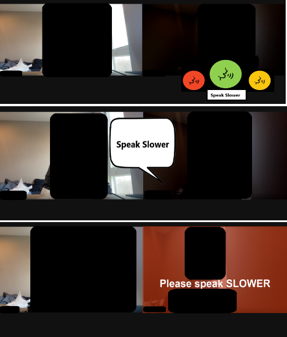
Critical Factors for Automatic Speech Recognition in Supporting Small Group Communication Between People who are Deaf or Hard of Hearing and Hearing Colleagues
Matt Huenerfauth, PI
National Science Foundation
To promote inclusion and success of D/HH employees in workplace communication, we investigate the use of Automatic Speech Recognition (ASR) technology for automatically providing captions for impromptu small-group interaction. We will conduct interviews with D/HH users, employers, and hearing co-workers; participatory design sessions and prototype usability testing with users; lab-based studies investigating how the presentation of ASR text output may influence the speaking behavior of hearing colleagues; experimental sessions with pairs or small groups of D/HH and hearing individuals collaborating on a problem solving-task while using a prototype ASR communication system; and observations of the use of prototype designs in real workplace settings. The project will result in human-computer interaction design and evaluation guidelines for the use of ASR in small group communication; broader impacts include societal benefits and STEM research opportunities for DHH students.
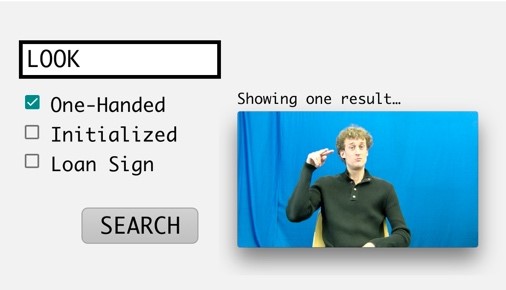
Scalable Integration of Data-driven and Model-based Methods for Large Vocabulary Sign Recognition and Search
Matt Huenerfauth, PI
National Science Foundation
Sign recognition from video is still an open and difficult problem because of the nonlinearities involved in recognizing 3D structures from 2D video, as well as the complex linguistic organization of sign languages. Purely data-driven approaches are ill-suited to sign recognition given the limited quantities of available, consistently annotated data and the complexity of the linguistic structures involved, which are hard to infer. Prior research has, for this reason, generally focused on selective aspects of the problem, often restricted to limited vocabulary, resulting in methods that are not scalable. We propose to develop a new hybrid, scalable, computational framework for sign identification from a large vocabulary, which has never before been achieved. This research will strategically combine state-of-the-art computer vision, machine-learning methods, and linguistic modeling. Looking up an unfamiliar word in a dictionary is a common activity in childhood or foreign-language education, yet I there is no easy method for doing this in ASL. The above framework will enable us to develop a user-friendly, video-based sign-lookup interface, for use with online ASL video dictionaries and resources, and for facilitation of ASL annotation. This research will (1) revolutionize how deaf children, students learning ASL, or families with deaf children search ASL dictionaries; (2) accelerate research on ASL linguistics and technology, by increasing efficiency, accuracy, and consistency of annotations of ASL videos through video-based sign lookup; and (3) lay groundwork for future technologies to benefit deaf users, e.g., English-to-ASL translation, for which sign-recognition is a precursor. The new linguistically annotated video data and software tools will be shared publicly.
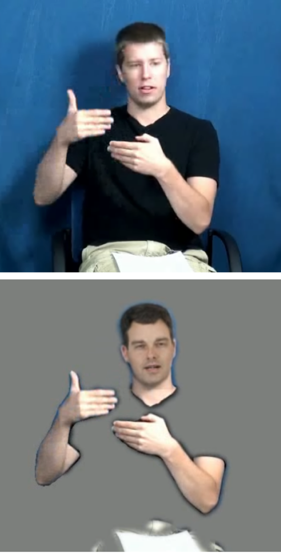
Data & AI Methods for Modeling Facial Expressions in Language with Applications to Privacy for the Deaf, ASL Education & Linguistic Research
Matt Huenerfauth, PI
National Science Foundation/Rutgers
This multi-university research project investigates robust artificial-intelligence methods for facial analytics that can be widely used across domains, applications, and language modalities (signed and spoken languages). This proposed work includes (1) extensions to ASL-based tools, AI methods, and data sharing; (2) an application to enable researchers to contribute video clips for analysis of facial expressions and head gestures; (3) an application to help ASL learners produce more accurately the types of facial expressions and head gestures that convey essential grammatical information in signed languages; and (4) a tool for real-time anonymization of ASL video communications, to preserve essential grammatical information expressed on the face/head in sign languages (SL) while de-identifying the signer in videos to be shared anonymously. Experimental user studies to assist in the in design of (3) and (4) will be conducted by Huenerfauth at RIT. Individuals who are Deaf and Hard of Hearing will be actively involved the research, including perceptual experiments to test comprehensibility and to investigate factors that influence acceptance of the ASL animations. This Phase I project lays the groundwork for a future Phase II proposal to the NSF Convergence Accelerator program. |
CAREER: Brain-inspired Methods for Continual Learning of Large-scale Vision and Language Tasks
Christopher Kanan, PI
National Science Foundation
The goal of this research project is to create deep neural networks that excel in a broad set of circumstances, are capable of learning from new data over time, and are robust to dataset bias. Deep neural networks can now perform some tasks as well as humans, such as identifying faces, recognizing objects, and other perception tasks. However, existing approaches have limitations, including the inability to effectively learn over time when data is structured without forgetting past information, learning slowly by looping over data many times, and amplification of pre-existing dataset bias which results in erroneous predictions for groups with less data. To overcome these problems, this research project aims to incorporate memory consolidation processes inspired by the mammalian memory system that occur both when animals are awake and asleep. The new methods developed in this project could lead to machine learning systems that 1) are more power efficient, 2) can learn on low-powered mobile devices and robots, and 3) can overcome bias in datasets. In addition, a significant educational component involves training the next generation of scientists and engineers in deploying machine learning systems that are safe, reliable, and well tested via new courses and programs.
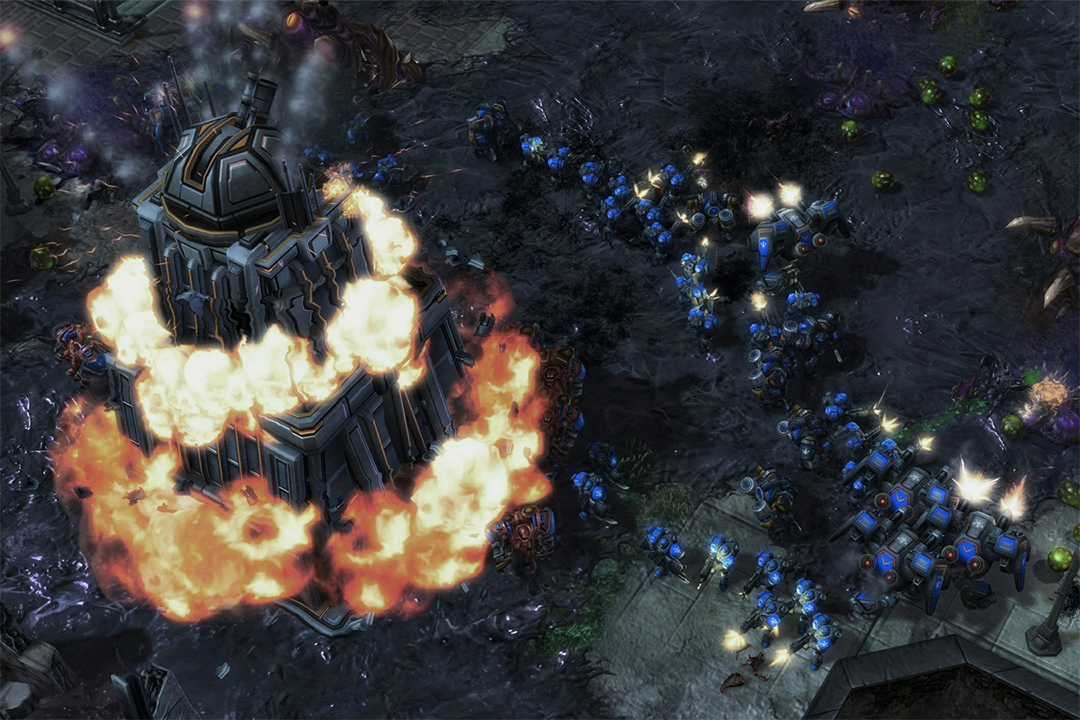
RI: Small: Lifelong Multimodal Concept Learning
Christopher Kanan, PI
National Science Foundation
While machine learning and artificial intelligence has greatly advanced in recent years, these systems still have significant limitations. Machine learning systems have distinct learning and deployment phases. If new information is acquired, the entire system is often rebuilt rather than having only the new information being learned because otherwise the system will forget a large amount of its past knowledge. Systems cannot learn autonomously and often require strong supervision. This project aims to address these issues by creating new multi-modal brain-inspired algorithms capable of learning immediately without excess forgetting. These algorithms can enable learning with fewer computational resources, which can facilitate learning on devices such as cell phones and home robots. Fast learning from multimodal data streams is critical to enabling natural interactions with artificial agents. Autonomous multimodal learning will reduce reliance on annotated data, which is a huge bottleneck in increasing the utility of artificial intelligence, and may enable significant gains in performance. This research will provide building blocks that others can use to create new algorithms, applications, and cognitive technologies.
The algorithms are based on the complementary learning systems theory for how the human brain learns quickly. The human brain uses its hippocampus to immediately learn new information and then this information is transferred to the neocortex during sleep. Based on this theory, streaming learning algorithms for deep neural networks will be created, which will enable fast learning from structured data streams without catastrophic forgetting of past knowledge. The algorithms will be assessed based on their ability to classify large image databases containing thousands of categories. These systems will be leveraged to pioneer multimodal streaming learning for visual question answering and visual query detection, enabling language. In Gallardo, Hayes, and Kanan (2021), we showed that self-supervised learning can greatly enhance online continual learning compared to supervised systems. This plot shows a REMIND system being updated with new data over time using self-supervised initialization. It learns 900 object categories incrementally in an online manner. It is orders of magnitude faster than other approaches, while also achieving state of the art results in terms of accuracy.
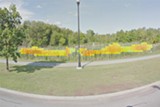
Detecting Invasive Species via Street View Imagery
Christopher Kanan, PI
Christy Tyler, Co-PI
NY Department of Environmental Conservation
Invasive plants are a significant economic and ecological problem globally, but manual monitoring is time consuming and resource intensive. We aim to use artificial intelligence (AI) to automatically detect the presence of target high-priority species in street level imagery. In preliminary work, we developed AI algorithms that excel at detecting two species that are invasive to New York State (NYS). In this project, we are proposing to extend the system to additional high-priority invasive species (e.g., Tree of Heaven) that pose significant economic and ecological risks to NYS. We propose to integrate our algorithms with NYSDEC’s iMap lnvasives system as a publicly available data layer to improve the ability to map out previously unknown infestations, track changes over time, and develop priority targets for containment and control efforts.
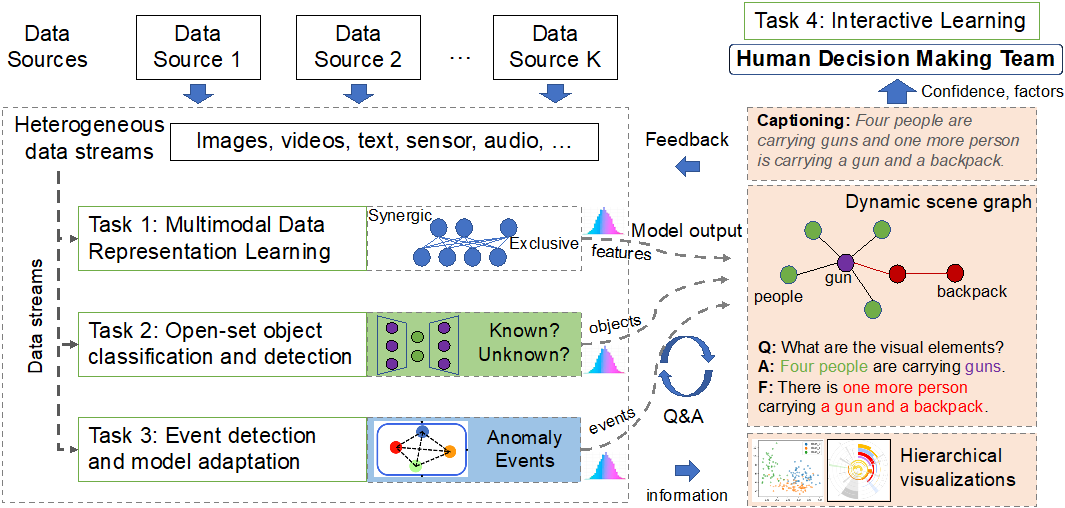
Dynamic Scene Graphs for Extracting Activity-based Intelligence
Yu Kong, PI
Qi Yu, Co-PI
DoD/ARO
Activity-based Intelligence (ABI) is an analysis methodology, which rapidly integrates data from multiple intelligence sources around the interactions of people, events, and activities, in order to discover relevant patterns, determine and identify change, and characterize those patterns to drive collection and create decision advantage. In this project, we plan to develop Dynamic Scene Graphs over large-scale multimodal time series data for representation learning. The new representation will enable learning from complex and dynamic environment, where a variety of vision tasks can be achieved including open-set object classification and detection, event detection, question-answering, and dense captioning.
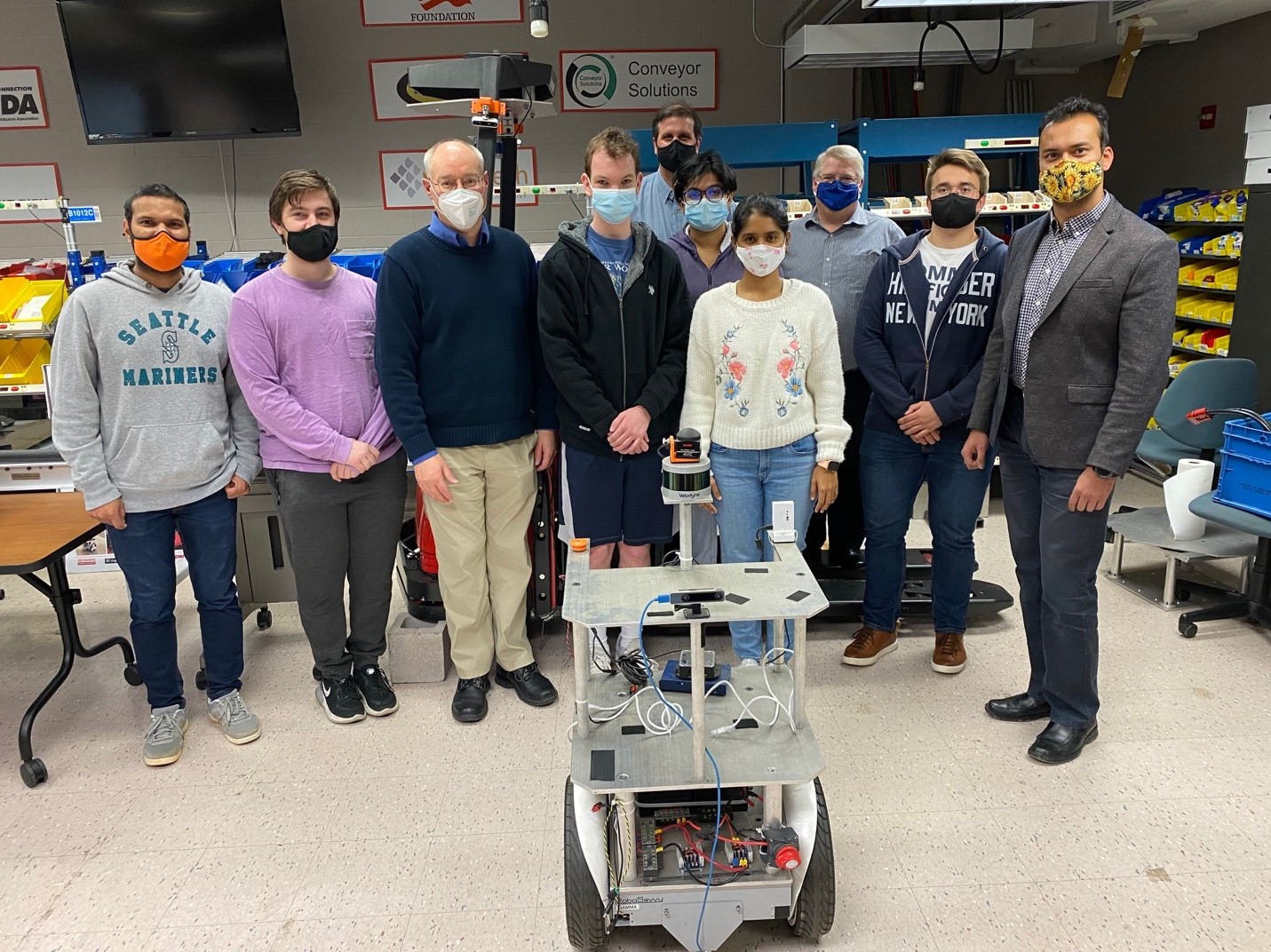
Effective and Efficient Driving for Material Handling
Michael Kuhl, PI
Amlan Ganguly, Co-PI
Clark Hochgraf, Co-PI
Andres Kwasinski, Co-PI
National Science Foundation
In warehousing operations involving a mix of autonomous and human-operated material handling equipment and people, effective and efficient driving is critical. We propose to address a set of integrated areas of research to enable improved real-time decision making leading to improved productivity, information, and communication. These include human-robot interaction/collaboration – avoiding incidents and improving predicted actions; and robust, low latency, secure vehicle to vehicle and system communication.
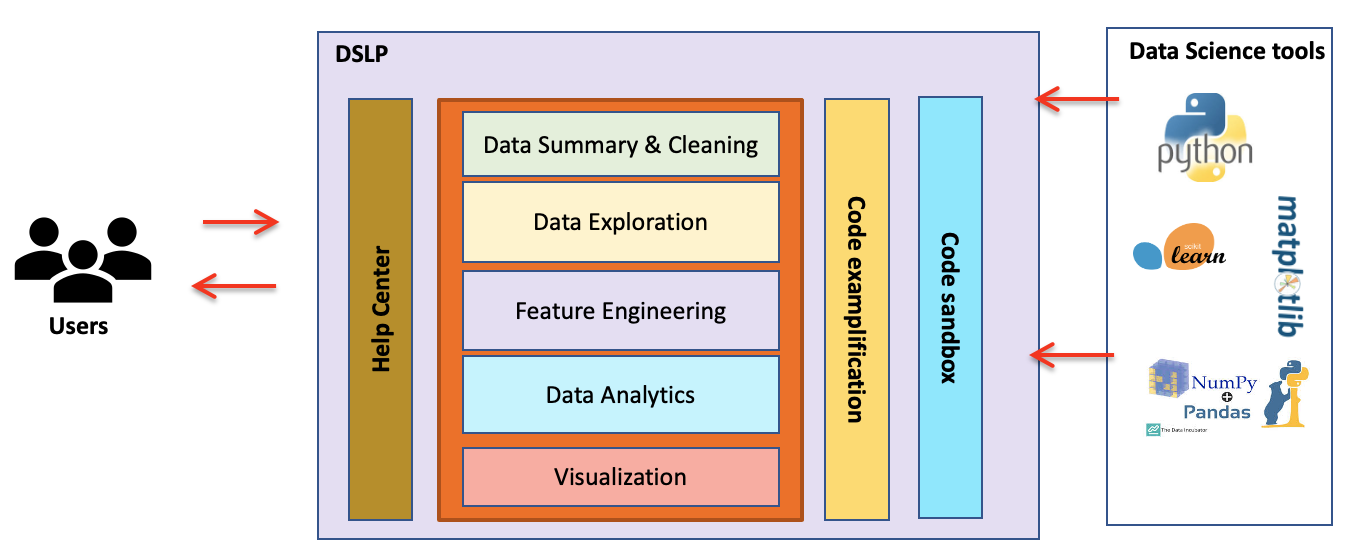
Developing a Hands-on Data Science Curriculum for Non-Computing Majors
Xumin Liu, PI
Erik Golen, Co-PI
National Science Foundation
Data science has become inherent across the spectrum and ramped up the demand for data science education across diverse disciplines. However, it is challenging for non-computing majors to access data science curriculum due to the long prerequisite chain of courses in programming, data structures, and introductory databases, as well as relevant mathematics. To address this challenge, this project proposes two deliverables, a web-based platform and an appropriate curriculum, to make hands-on data science accessible for non-computing majors, regardless of their programming and mathematical background. More importantly, these students will learn to use data science techniques in the context of their own disciplines, rather than first become computer scientists and subsequently data scientists. The non-computing majors covered by the project are those might have taken a high school AP Computer Science Principles (CSP) class or an equivalent CSP course increasingly offered in many colleges, and will take a follow-on Data Science Principles (DSP) course with materials developed by the project.
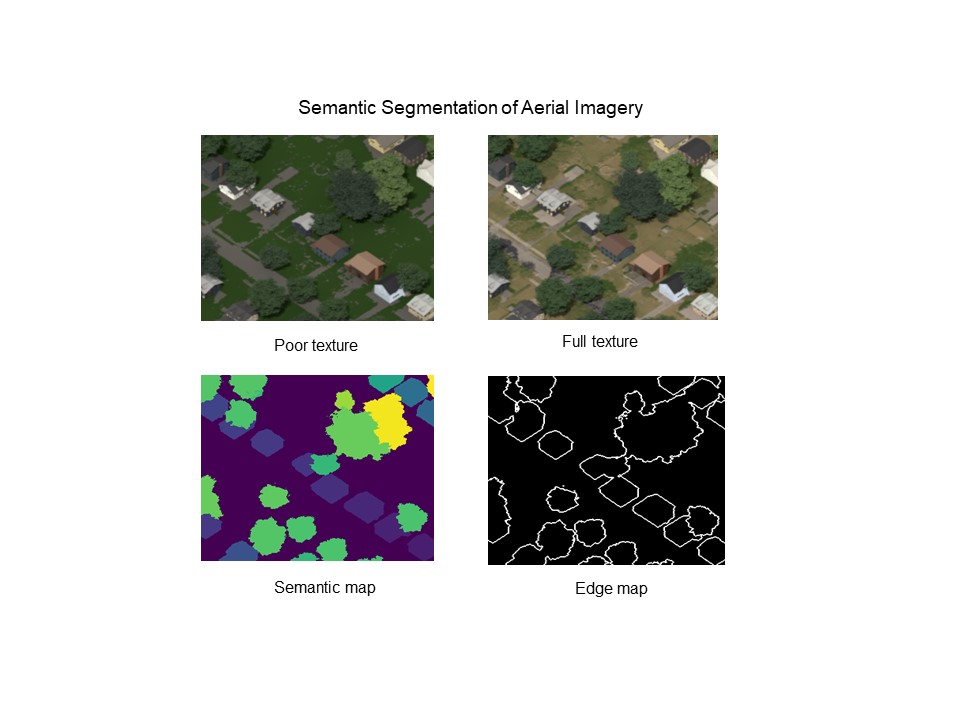
Trilobyte – Autonomous Learning for Full-Spectrum Sims
Alexander Loui, PI
Carl Salvaggio, Co-PI
L3 Harris Technologies
This multi-year project aims to develop an end-to-end deep learning solution to generate representative full-spectrum 3D point clouds from single-band imagery, thereby improving the efficiency and accuracy in building full-spectrum synthetic scenes using Digital Imaging and Remote Sensing Image Generation (DIRSIG). The scope of the project includes multimodal data collection as well as development of deep learning-based 3D registration and semantic segmentation algorithms. It also includes generating corresponding DIRSIG scenes and accompanying synthetic image data for training.
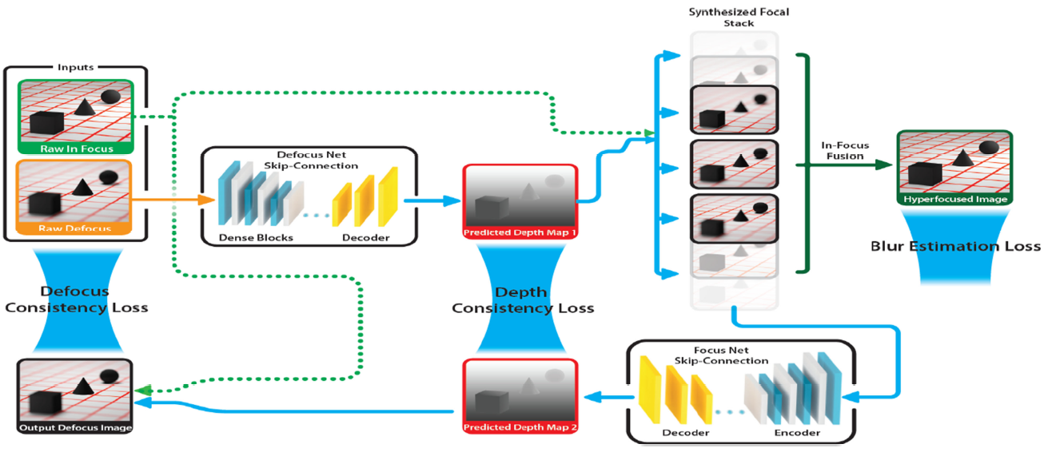
Collaborative Research: SHF: Small: Enabling Efficient 3D Perception: An Architecture-Algorithm Co-Design Approach
Guoyu Lu, PI
National Science Foundation
The objective of the proposed research is to rethink the systems stack, from algorithms to hardware, for 3D perception, i.e., point cloud processing, so as to enable 3D perception as a fundamental building block in emerging domains such as autonomous driving, Augmented/Virtual Reality, and smart agriculture.
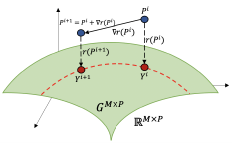
Collaborative Research: CDS&E: Theoretical Foundations and Algorithms for L1-Norm-Based Reliable Multi-Modal Data Analysis
Panos Markopulous, PI
Andreas Savakis, Co-PI
National Science Foundation
This project focuses on providing theoretical foundations and algorithmic solutions for reliable, L1-norm-based analysis of multi-modal data (tensors). The proposed research is organized in three main thrusts. In Thrust 1, we will focus on investigating theoretically (e.g., hardness and connections to known problems) and solving L1-norm-based Tucker and Tucker2 decompositions (L1-TUCKER and L1-TUCKER2, respectively). In Thrust 2, we will focus on developing efficient (i.e., low-cost, near-optimal) and distributed solvers for L1-TUCKER and L1-TUCKER2, appropriate for the analysis of big data and data in the cloud. In Thrust 3, we will investigate the application of the developed algorithms to tensor analysis paradigms from the fields of computer vision, deep learning, and social-network and stock-content data analytics. Overall, this project aspires to provide algorithmic solutions that will support reliable data-enabled research in a plethora of disciplines across science and engineering.
Towards Adversarially Robust Neuromorphic Computing
Cory Merkel
Air Force Research Laboratory
The recent artificial intelligence (AI) boom has created a growing market for AI systems in size, weight, and power (SWaP)-constrained application spaces such as wearables, mobile phones, robots, unmanned aerial vehicles (UAVs), satellites, etc. In response, companies like IBM, Intel, and Google, as well as a number of start-ups, universities, and government agencies are developing custom AI hardware (neuromorphic computing systems) to enable AI at the edge. However, many questions related to these systems' security and safety vulnerabilities still need to be addressed. Since the early 2000's a number of studies have shown that AI and especially deep learning algorithms are susceptible to adversarial attacks, where a malicious actor is able to cause high-confidence misclassifications of data. For example, an adversary may easily be able to hide a weapon from an AI system in plain sight by painting it a particular color. A number of defense strategies are being created to improve AI algorithm robustness, but there has been very little work related to the impact of hardware-specific characteristics (especially noise in the forms of low precision, process variations, defects, etc.) on the adversarial robustness of neuromorphic computing platforms. To fill this gap, we propose the following research objectives: Objective 1. Evaluate and model the effects of noise on the susceptibility of neuromorphic systems to adversarial attacks. Objective 2. Design adversarially-robust training algorithms for neuromorphic systems. Objective 3. Develop novel adversarial attacks that leverage hardware-specific attributes of neuromorphic systems.
CAREER: A Computational Approach to the Study of Behavior and Social Interaction
Ifeoma Nwogu, PI
National Science Foundation
In this work, the classical social challenge of studying the interactive behaviors of humans in a group is cast as one of computationally modeling the collective properties of multiple interacting, dynamic multimodal signals in a network. We aim to develop a comprehensive set of network analysis methods that use Bayesian trained neural networks to learn the constantly changing structural patterns of a social network, which itself is made up of interacting temporal signals. In this five-year proposal, our integrated research, education and outreach goals aim to: (i) collect and collate data generated in small face-to-face, complex, real-life contexts; (ii) develop new, interpretable, dynamic neural network models, to evaluate the collective properties of constantly changing graphs that represent how people in small social groups interact with one another over time; (iii) form a global consortium of academic participants (researchers, graduate and undergraduate students) that have a set of core competencies in artificial intelligence, computer vision and human language research, with the specific goal of supporting underrepresented STEM groups.
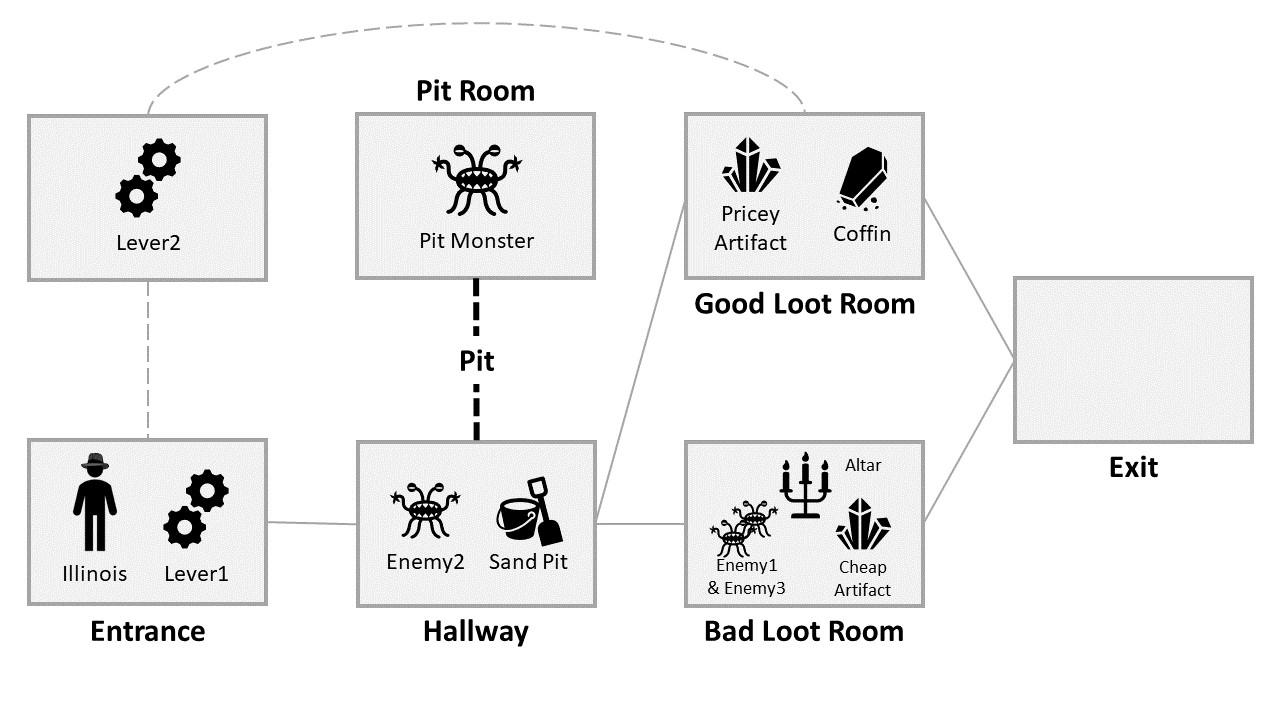
A Deep Gameplay Framework for Strong Story Experience Management
Justus Robertson, PI
National Science Foundation
Experience managers are intelligent agents that produce personalized stories that change based on decisions a player makes in a digital game. These agents create new and powerful types of interactive stories for art and entertainment, training applications, and personalized education. A central problem for experience managers is avoiding dead ends, which are situations where story structure is broken due to a choice by the player. This problem results in experiences with un-interesting stories, missed training sequences, and long spells without appropriate targeted educational content. This project will develop a novel experience management architecture to quickly navigate around dead end situations during real-time interaction with a human participant. The architecture is based on recent advances in deep reinforcement learning for general game playing. This experience management platform will enable new forms of real-time training and education applications.
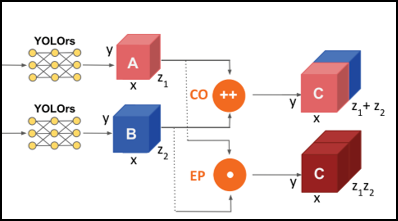
Target Detection/Tracking and Activity Recognition from Multimodal Data
Eli Saber, PI
Jamison Heard, Co-PI
National Geospatial-Intelligence Agency
Our primary objectives in this proposal are to develop operational target detection/tracking techniques and activity recognition/understanding methods that possess the capability to leverage multimodal data in a fusion framework using deep learning algorithms and coupled tensor techniques while providing accurate and near real-time performance. The proposed methodology is divided into four major stages: (1) pre-processing, (2) co-registration and fusion, (3) target detection and tracking, and (4) activity recognition and scene understanding. The aforementioned algorithms will be benchmarked against existing state of the art techniques to highlight their advantages and distinguish their abilities. The above is intended to assist analysts to effectively and efficiently ingest large volumes of data, or perform object detection and tracking in real-time under dynamic settings. |
Data-Driven Adaptive Learning for Video Analytics
Andreas Savakis, PI
Christopher Kanan, Co-PI
Panos Markopoulos, Co-PI
Air Force Office of Scientific Research
The objective of this research project is to design a Data Driven Adaptive Learning framework for visual recognition. The adaptive nature of this framework is suitable for recognition in new domains, that are different from those used for training, and in data starved environments where the number of available training samples is small. We design classification engines that learn incrementally, without full retraining, using continuous updating methods as new data become available. These adaptive learners will incorporate weakly labeled data as well as human in the loop to facilitate annotation of previously unseen data, and will adapt to new environments dynamically in cooperation with multimodal sensors and a dynamic control unit. We design, implement and test the following classifier engines as they have high potential to operate effectively within our framework: a) incremental subspace learning using robust techniques and related applications to classification and adaptation to new domains; b) adaptive deep learning and applications to recognition of people, vehicles, etc.
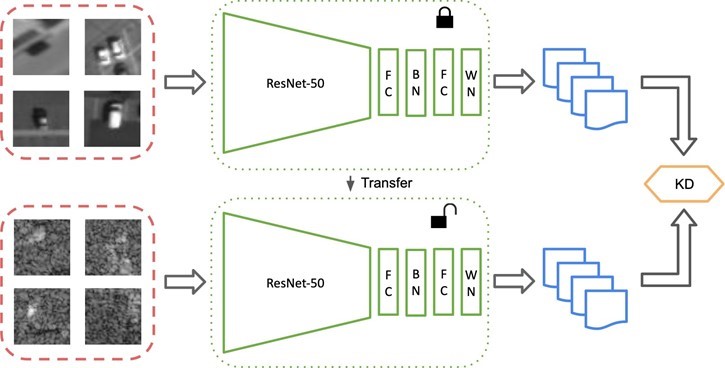
Multimodal Sensing, Domain Adaptation and Transfer Learning
Andreas Savakis, PI
USAF SBIR (with IFT)
The proposed project is to extend basic research in contemporary areas that supports the vision of artificial intelligence (AI) autonomy for real time operational support. AI for computer vision consists of Deep Learning and Machine Learning from which typical examples focus on multi-media content such as imagery; but basic research theory elements of joint multimodal DL have yet to be realized for intelligence, surveillance, and reconnaissance applications such as electro-optical (EO) and synthetic aperture radar (SAR). Additionally, there is a need to transfer from one domain (e.g., SAR) to another domain (e.g. EO) in contexts were data availability is limited.
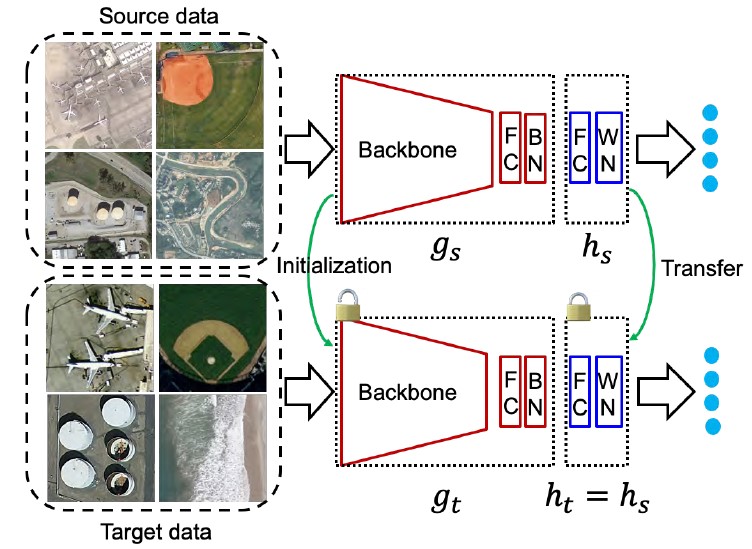
Global Surveillance Augmentation Using Commercial Satellite Imaging Systems
Andreas Savakis, PI
USAF SBIR (with Kitware Inc.)
NYS/UR Center for Emerging and Innovative Systems (CEIS)
The proposed project is to extend basic research in contemporary areas that supports the vision of artificial intelligence (AI) autonomy for real time operational support. AI for computer vision consists of Deep Learning and Machine Learning from which typical examples focus on multi-media content such as imagery; but basic research theory elements of joint multimodal DL have yet to be realized for intelligence, surveillance, and reconnaissance applications such as electro-optical (EO) and synthetic aperture radar (SAR). Additionally, there is a need to transfer from one domain (e.g., SAR) to another domain (e.g. EO) in contexts were data availability is limited. |
Peri-procedural transmural electrophysiological imaging of scar-related ventricular tachycardia
Linwei Wang, PI
DHHS/National Institutes of Health
The majority of life-threatening ventricular arrhythmia episodes are caused by an electrical "short circuit", formed by a narrow channel of surviving tissue inside myocardial scar. An important treatment is to target and destroy these culprit slow-conducting channels. Unfortunately, with conventional catheter mapping, up to 90% of the VT circuits are too short-lived to be mapped. For the 10% “mappable” VTs, their data are available only during ablation and limited to one ventricular surface. This inadequacy of functional VT data largely limits our current ablation strategies. The goal of this proposal is to develop a novel technique to provide pre- and post-ablation functional arrhythmia data – integrated with LGE-MRI scar data in 3D – to improve ablation with pre-procedural identification of ablation targets and post-procedural mechanistic elucidation of ablation failure. Its specific objectives include: 1) To develop and validate a peri-procedural electrocardiographic imaging (ECGi) technique for mapping scar-related VT circuits. 2) To integrate LGE-MRI scar into ECGi for improved electroanatomical investigations of VT circuits. 3) To perform clinical evaluation of pre-ablation and post-ablation MRI-ECGi of scar-related VT. This project is carried out in collaboration with Siemens Healthineers, Nova Scotia Health Authority, and University of Pennsylvania.
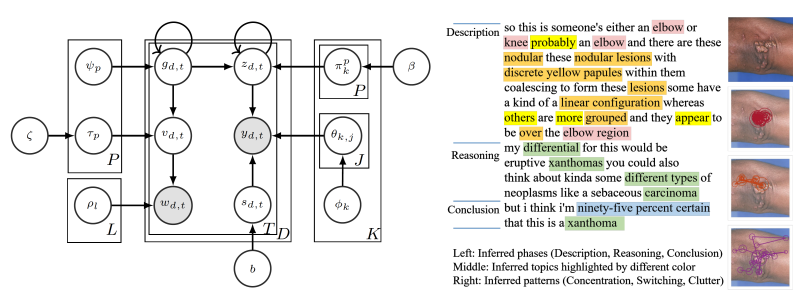
Utilizing Synergy between Human and Computer Information Processing for Complex Visual Information Organization and Use
Qi Yu, PI
Anne Haake, Co-PI
Pengcheng Shi, Co-PI
Rui Li, Co-PI
National Science Foundation
This project will research computational models to represent different elements in human knowledge, aiming to understand how their interaction with image data underlies human image understanding. The modeling outcomes will inform algorithmic fusion of human knowledge with image content to create novel representations of image semantics as a result of human-machine synergy.
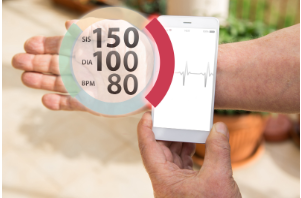
Measurement and relationship of physiological arousal and stress in children with ASD and caregivers
Zhi Zheng, PI
Peter Bajorski, Co-PI
NIH/UR Center for Emerging and Innovative Systems (CEIS)
The proposed research is a cross-sectional evaluation of the bidirectional relationship between parent and child arousal in dyads that include a child with autism spectrum disorder (ASD) and their primary caregiver. We propose the use of a wearable device that captures physiological stress data in parent/child dyads. Caregivers will complete diaries to provide context to specific events during which arousal and stress are likely to be high. The focus on the multiple factors that influence caregiver health will help meet both current and future health care needs in this population, which to date are largely unexplored. RIT researchers will be responsible for the configuration of physiological sensors, physiological data processing and analysis, as well as statistical analysis for the whole project.