Home Page
We all share the same reality, yet we each experience it differently. Solving problems collectively is complicated, and poses legitimate ethical concerns, yet collective solutions can be more robust, novel, and inclusive than those solved individually.
The same is true for artificial intelligence. Our lab designs and investigates algorithms that engage, model, and learn from human populations, creating dynamic systems that represent diverse values and beliefs, build trust, and advance community values in public policy and decision making.
Research
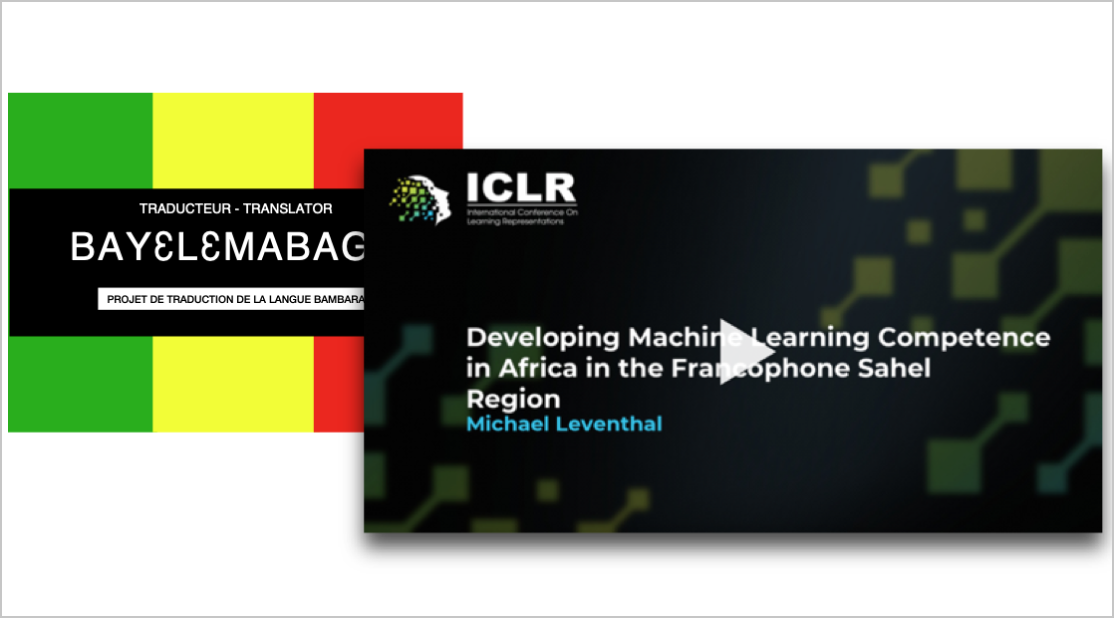
Bayelemabaga
We are developing methods to collect, clean data and evaluate and train translation models using crowdsourcing. And are striving to become a national project in Mali as part of its initiative to use science and technology to advance its education and economic development.
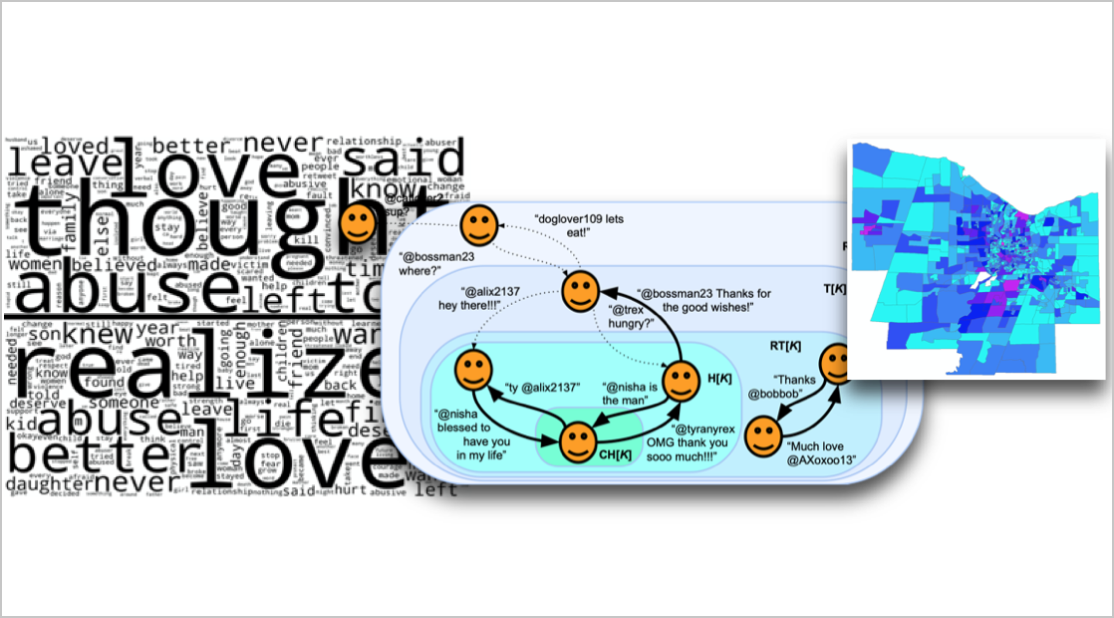
Predicting public health risks from first-person narratives
Health and well-being, particularly on a public scale depends greatly on our actions, social and otherwise. Many people around the world spend substantial periods of their lives online.
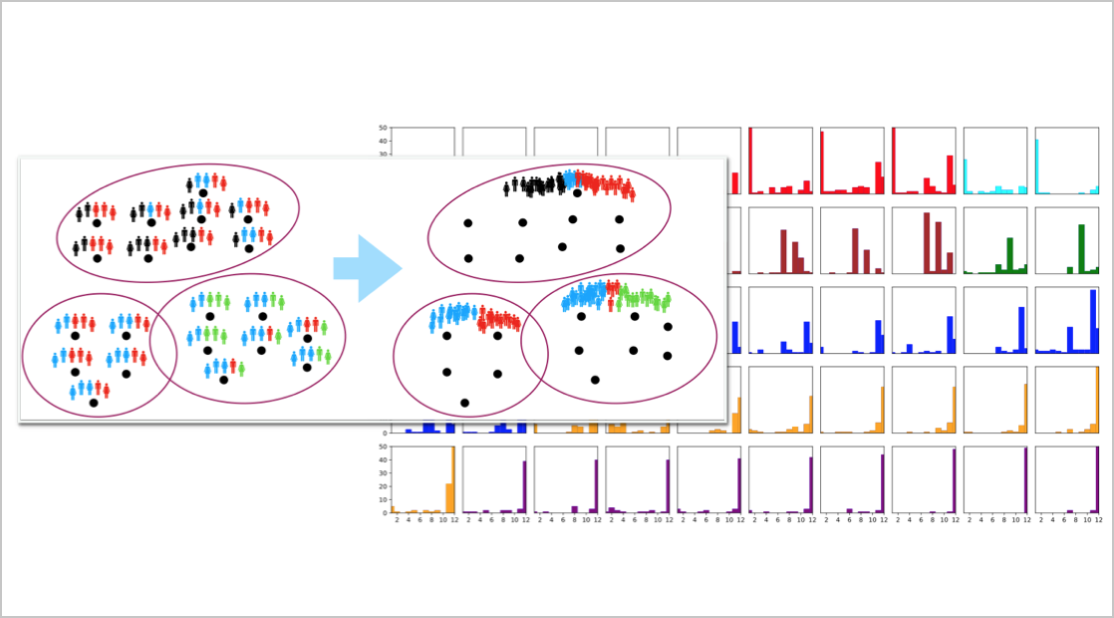
Diversity-preserving supervised learning
This project addresses basic problems that underlie the our lab’s more application-driven activities.
Our People
Christopher Homan, Ph.D.
Associate Professor
Computer Science
Golisano College of Computing and Information Sciences
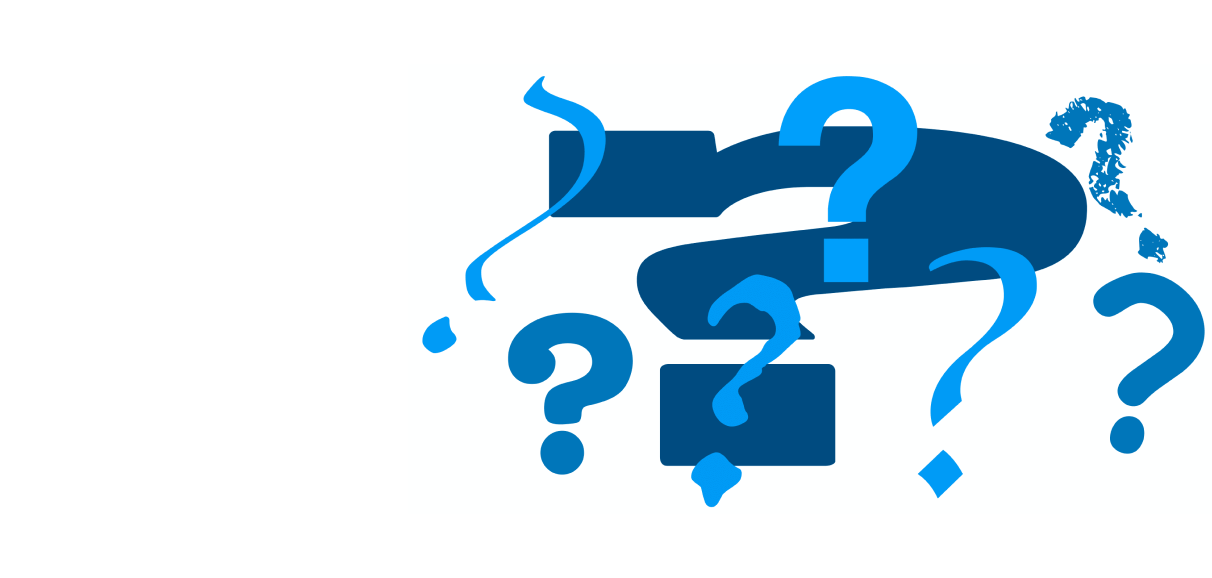