Perception for Movement Lab
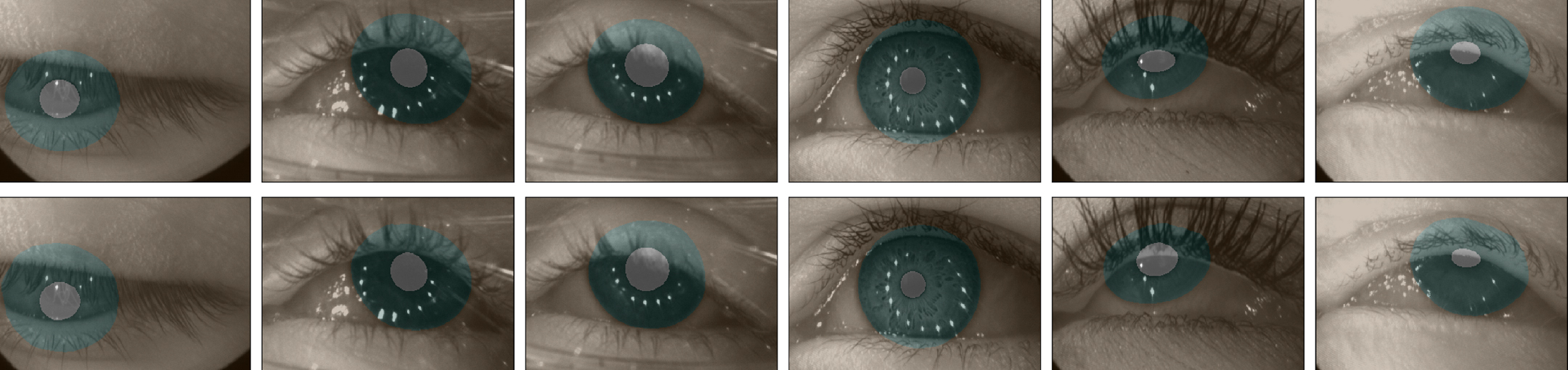
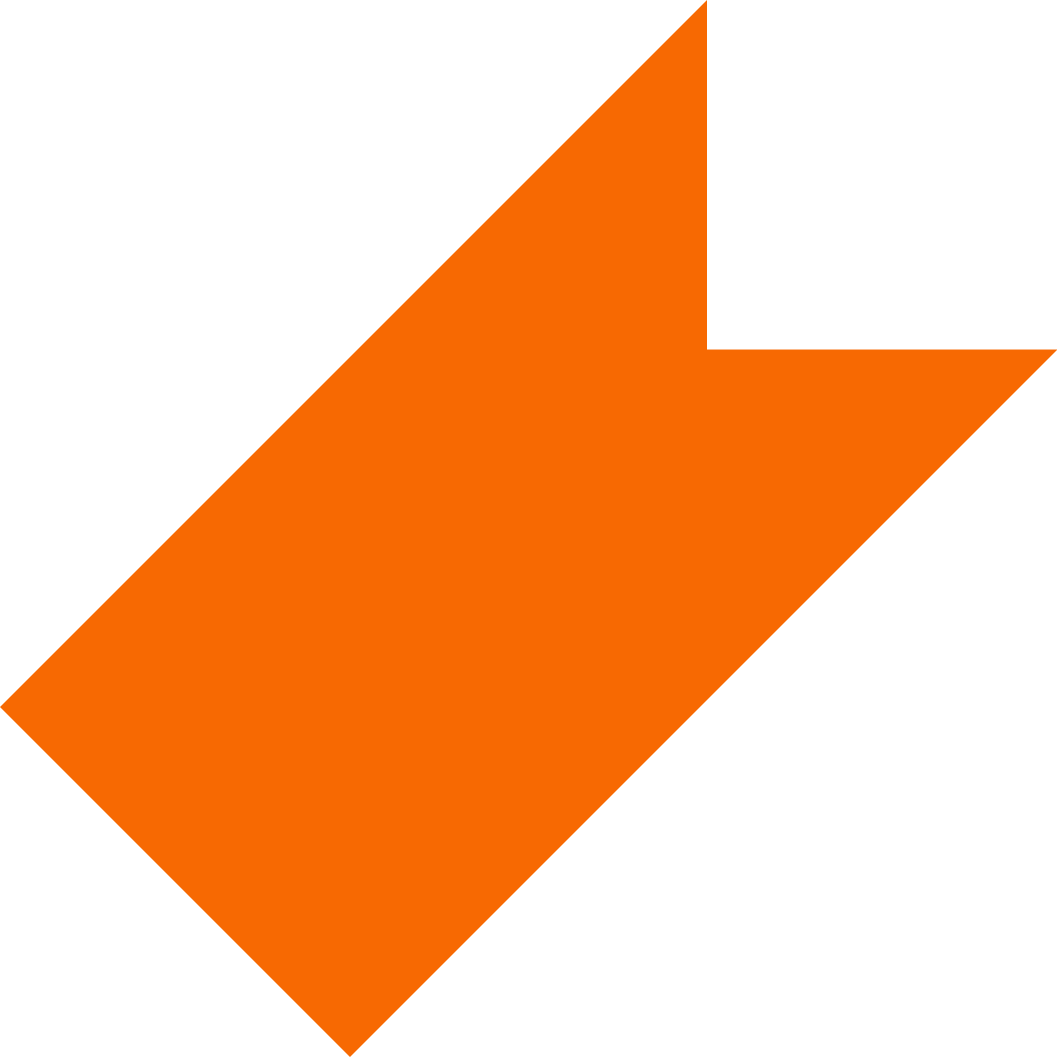
Perception For Movement Lab
Dedicated to bettering our understanding of the mechanisms that allow humans to perform everyday visually guided actions.
Overview
The PerForM (Perception For Movement) Lab is a part of the Multidisciplinary Vision Research Laboratory, and is dedicated to bettering our understanding of the mechanisms that allow humans to perform everyday visually guided actions, like catching a ball, driving a car, or walking over uneven terrain. Although these are natural actions, the natural environment does not afford the experimental control required for the rigorous testing of hypotheses about how vision is used to guide action. In contrast, traditional laboratory-based study has a tendency to validate hypothesis that are unique to the laboratory environment, and unrepresentative of real-world behavior. To resolve this conflict, the PerForM Lab both leverages and engineers advances in virtual reality and motion capture technologies that afford both freedom of movement, and a naturalistic visual experience. Using these tools, we are able to conduct controlled, naturalistic studies of the human visual and motor systems.
Lab Members
Direct Advisees
Catherine Fromm, Ph.D. candidate in CIS (LinkedIn)
Undergraduate Degree in Physics and Music (2016) - Wellesley College
Arianna Giguere, Ph.D. candidate in CIS (LinkedIn)
Undergraduate Degree in Physics, with a minor in Mathematics (2019) – University of Maine
Abhijan Wasti, M.S. candidate in CIS (personal webpage)
Kevin Barkevich, Ph.D. candidate in Computing and Information Science (LinkedIn)
Student collaborators
Chengyi Ma, M.S. candidate in Computing and Information Science (LinkedIn)
Jackson Shuminski, M.S. candidate in Computing and Information Science (LinkedIn)
Kamran Binaee, Ph.D. Imaging Science 2019 (LinkedIn. Google Scholar)
Rakshit Kothari, Ph.D. Imaging Science 2021 (LinkedIn. Google Scholar)
Current Projects/Demos
Collaborators: Jeff B. Pelz (Imaging Science @ RIT) and Reynold R. Bailey (Computer Science @ RIT)
Supported by: Meta/Facebook
A mobile eye tracker typically has the form factor of a pair of sunglasses, with three integrated cameras: two near-eye infrared cameras directed towards the observer's eyes, and one outward-facing scene camera on the observer's forehead. The process of gaze estimation occurs mostly in the form of software algorithms that form a mapping between image features detected in each eye camera, such as the centroid of the detected pupil, to a location on the scene camera. Inferences about gaze behavior are made by drawing this estimated gaze as a crosshair upon the 2D scene camera imagery or, through additional computation, as a ray cast from the eye into a digital model of the surrounding 3D environment. Critically, the robustness, precision, and accuracy of this estimate rely heavily upon the quality of the intervening step of detecting features in the 2D eye imagery.
Our laboratory has been pioneering new methodologies using machine learning for the segmentation of eye imagery into pixels that represent the pupil, iris, sclera, and “other” pixels. These segmentations are extremely accurate and robust to intervening optics (e.g. glasses or contacts), the presence of makeup, and partial occlusion from the eyelids. Our 2019 model, RITNet, won first place in Facebook Reality Labs’ OpenEDS segmentation challenge. Examples of the application of one of more recent networks to the 2019 OpenEDS dataset can be seen below.
Collaborators: Krystel Huxlin (University of Rochester), and Ross Maddox (University of Rochester)
Supported by: National Institutes of Health: National Eye Institute 1R15EY031090
Stroke-induced occipital damage is an increasingly prevalent, debilitating cause of partial blindness, which afflicts about 1% of the population over the age of 50 yrs. Until recently, this condition was considered permanent. Over the last 10 years, the Huxlin lab has pioneered a new method of retraining visual discriminations in cortically blind (CB) fields. The Perform Lab is currently working with Drs. Huxlin and Maddox to address known limitations by integrating precision eye tracking into a modern VR helmet, and porting the CB visual retraining paradigm into this system.
This approach aims to improve the efficacy and practicality of these pioneering visual training methodologies so that training may be conducted at home, using stimuli presented in virtual reality. At the same time, we are testing new training methodologies that leverage the 3D spatial properties of virtual reality displays, and the availability of low-cost eye trackers integrated into the head mounted display for gaze-contingent stimulus delivery to the blind portion of the observer’s field of view. This work recognizes that the use of passive, single-target-on-a-uniform-background stimulus delivery requiring only a perceptual judgment may not be the most effective way to retrain vision in CB. An alternative notion is that additional cues present in the real world (e.g. sound, depth, color and target-in-background context) may improve the quality and reduce the timing of training required before for improvement.
When preparing to intercept a ball in flight, humans make predictive saccades ahead of the ball’s current position and to a location along the ball’s future trajectory. Such visual prediction is ubiquitous amongst novices, extremely accurate, and can reach at least 400ms into the future. This ocular predicion is not a simple extrapolation of a ball’s trajectory. Previously, this was demonstrated in a virtual-reality ball interception task, in which subjects were asked to intercept an approaching virtual ball shortly after its bounce upon the ground. Because movement of the body was tracked with a motion-capture system, subjects were able to affect a virtual ball seen through a head-mounted display. Subjects made spontaneous pre-bounce saccades to a location along the ball’s eventual post-bounce trajectory, where they would fixate until the ball passed within 2˚ of the fixation location. Importantly, the saccades also demonstrated accurate prediction of the ball’s speed in the vertical direction after the bounce, and thus the eventual height of the ball at the time of the catch.
Humans in the natural environment are able to maintain efficient and stable gait while navigating across complex terrain. In part, this is because visual feedback is used to guide changes in heading, foot placement, and posture. However, the role of gaze-adjustments and the role of visual information in heading selection and postural control while walking is not well understood.
We present a novel method for the study of visually guided walking over complex terrain in a laboratory setting. A ground-plane is projected upon a physically flat laboratory floor in a way that makes it appear to have height, similar to a one-plane CAVE environment. The percept of height is reinforced through the use of stereoscopic shutter glasses, and by the dynamic updating of the projected image to maintain the coincidence of the accidental perspective with the subject’s motion-tracked head position. The benefits of this “phantogram” ground-plane are numerous. Obstacle height, shape, and location can be instantaneously manipulated, decreasing setup time, and increasing the number of trials-per session. Because the ground-plane is physically flat, the likelihood of falls is dramatically reduced. Furthermore, because the use of whole-body motion capture, eye trackers, and computer imagery provides a record of the position of the head, body, and gaze in relation to objects in the scene, the environment facilitates the computational analysis of eye-tracking data in relation to postural control during locomotion. Furthermore, the apparatus offers the possibility of real-time manipulations of the environment that are contingent upon the participant’s behavior. In summary, the apparatus facilitates rigorous hypotheses testing in a controlled environment.
This work is being conducted in coordination with fellow collaborators Brett Fajen (RPI), Melissa Parade (RPI), Jon Matthis (UT Austin), and Mary Hayhoe (UT Austin).
Some important questions:
- Is this a valid method for studying “natural” walking behavior?
- What does gaze tell us about how information is used to guide postural control while walking?
- What do eye-movements tell us about the degree to which postural control and foot placement rely upon memory of obstacle location?
Previous Projects
Collaborators: Jeff Pelz, Reynold Bailey, and Chris Kanan at RIT
Sponsors: Google, Inc.
The future of virtual and augmented reality devices is one in which media and advertising is seamlessly integrated into the 3D environment. Now that the user's visual attention is freed from the confines of the traditional 2D display, new advances will be required to detect when a user is visually attending to an object or advertisement placed in the 3D environment. One promising tool is eye tracking. However, identifying gaze upon a region of interest is particularly challenging when the object or observer are in motion - as is most often the case in the natural or simulated 3D environments. This is a challenging problem because the eye-tracking community remains primarily constrained to the context of 2D displays, and has not produced algorithms suitable for the transformation of a 3D gaze position signal into a usable characterization of the subject's intentions.
Our lab is currently working to novel machine learning classification tool that analyzes movements of the head, and eyes for the automated classification of coordinated movements, including fixation, pursuit, saccade, whether they arise from movements of the eyes, head, or coordinated movements of both.
Graduate student Rakshit Kothari wears his custom hardware: a helmet that tracks the movements of the eyes and head when coordinated by task. This hardware involves a Pupil Labs eye tracker, inertial measurement unit, and stereo RGB cameras
A custom Matlab program allows a group of trained professionals to find and label fixations, saccades, and pursuit in the eye+head velocity signal.
Decades of research upon eye movements made in the laboratory have produced a firm understanding of the parameters of eye movements, including duration, dispersion, amplitudes, and velocities. No such understanding exists for coordinated movements of the eyes and head made in the wild. In part, this is because algorithms for the analysis of gaze within the 3D environment are in their infancy. The lack of insight into gaze parameters and event statistics may be largely attributed to limitations in algorithmic tools for analysis of gaze behavior in 3D environments. Because an eye tracker reports only the orientation of the eyes within their orbit, existing algorithms for the classification of gaze events developed for the 2D context do not generalize well to the 3D context in which the head is free to move. For example, in the natural environment, one can maintain "fixation" of a target despite a constantly changing eye-in-head orientation through a counter-rotation of the eyes (vesibulo-ocluar reflex; VOR). To properly detect VOR other head+eye gaze events requires that movements of both the head and eye are taken into account. Our laboratory is solving these problems using custom technology, and the machine learning algorithms currently under development for the automated detection of eye movements.
Preliminary data shows the main sequence for gaze shifts made by an unconstrained behaving human.
Collaborators: Jeff Pelz (RIT)
Sponsors: The Federal Reserve and National Academy of Sciences
This study is designed to investigate the effectiveness of security features (e.g. watermark, ribbon, etc.) as cashiers interact with potentially counterfeit bills in natural workplace environment. The primary objectives are to 1) develop a methodology to robustly record the physical behaviors (e.g., actions and gestures) and visual attention of cashiers and customers during cash transactions, 2) develop a methodology to reliably analyze the physical behaviors and visual attention of cashiers and customers during cash transactions, 3) develop a methodology to robustly record the physical behaviors and visual attention of cashiers during currency handling (i.e., counting and sorting) tasks, 4) develop a methodology to reliably analyze the physical behaviors of cashiers during currency handling tasks, and 5) to use those methods to collect data on a sample of customers and enough cashiers to explore variation due to age and experience.
Equipment
We have a collection of virtual reality helmets with integrated eye trackers, including:
- Several HTC Vive Pro with Pupil Labs eye tracking Integration
- Several HTC Vive Eye Pro (with eye tracking integration by Tobii)
- Mobile eye trackers by Pupil Labs
- Several Pupil Invisible mobile eye trackers
- A 14 Camera Phasespace Motion capture system that spans a 7.5m by 5.5m capture volume with a 980 Hz refresh rate. The end-to-end latency from motion-detection to visual updating of a minimal virtual environment viewed on a CRT display has been measured to be approximately 27 ms.
Publications
For the latest publications coming out of PerForm Lab, visit Gabriel J. Diaz's page on Google Scholar.