CHAI Research Talk: Dimah Dera PhD, Assistant Professor, Chester F. Carlson Center for Imaging Science, RIT
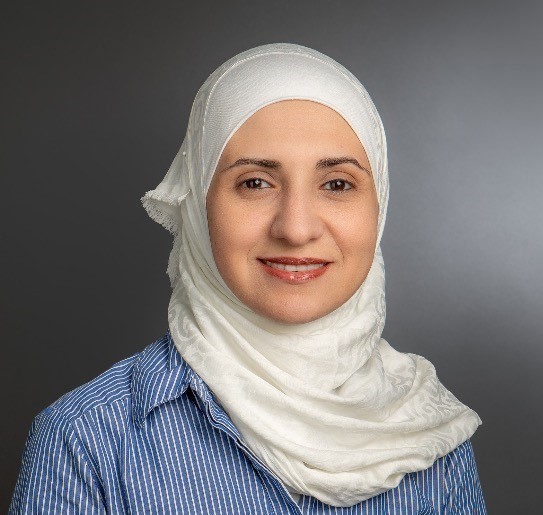
CHAI Fall 2024 Seminar Series Research Talk
Refreshments will be served
DATE: Monday, September 9, 2024, 3:00-4:00 PM
SPEAKER: Dimah Dera PhD, Assistant Professor, Chester F. Carlson Center for Imaging Science, Rochester Institute of Technology
TITLE: Robustness and Reliability of Machine Learning and
Its Importance in Imaging
IN PERSON: Golisano Hall (070), Room CYB-1710/1720
HOST: Cecilia O. Alm, PhD, Associate Director, CHAI; Professor, Department of Psychology; Joint Program Director, MS Program in Artificial Information, School of Information; Director, AWARE-AI NSF Research Traineeship Program; Director, Computational Linguistics and Speech Processing Lab
ABSTRACT: Machine learning algorithms, particularly deep neural networks, have gained significant prominence due to their success, especially in the fields of imaging science and computer vision. However, the question of how reliable these models are in various applications remains a critical challenge. The robustness and reliability of machine learning algorithms refer to a model's ability to maintain performance across diverse and challenging conditions, including noisy data, adversarial attacks, and domain shifts. Imaging data is inherently complex, with variations in lighting, scale, viewpoint, and heterogeneity. Robust models are essential to delivering consistent and accurate results, particularly in fields like medical imaging, autonomous vehicles, and surveillance systems. This presentation explores the concept of robustness and reliability of machine learning, focusing on its pivotal role within the realm of imaging science. We will shed light on the paramount importance of uncertainty quantification in bolstering the robustness of machine learning models. Uncertainty quantification is the process of estimating the confidence or uncertainty associated with model predictions. We will present state-of-the-art models for quantifying uncertainty and empowering the robustness of modern machine learning models towards real-world deployment in various applications.
BIO: Dimah Dera specializes in robust and trustworthy modern machine learning (ML) solutions for real-world applications, including healthcare, cyber- security, and surveillance systems. In the rapidly evolving landscape of artificial intelligence (AI) and autonomous systems, the integration of ML techniques has paved the way for unprecedented advancements across various domains. The scope of her research includes developing innovative techniques to ensure the robustness and reliability of AI systems by integrating Bayesian theory and statistical signal processing foundations into modern ML frameworks. This research highlights the intricate connections between learning Bayesian uncertainty in ML models and their robustness and safety awareness to dynamically changing environments and systems failure. Dimah received the NSF Computer and Information Science and Engineering Research Initiation Initiative (CRII) in 2023. She won multiple awards, such as the Best Paper Award at the 2019 IEEE International Workshop on Machine Learning for Signal Processing and the IEEE Philadelphia Sections Benjamin Franklin Key Award (2021). She publishes in the area of trustworthy, reliable, and expandable ML, signal and image processing and optimization.
NOTE: Access services (interpreter) have been requested for this event.
Event Snapshot
When and Where
Who
Open to the Public
Cost | FREE |
Interpreter Requested?
Yes