Imaging Science Ph.D. Defense: Applying Machine Learning to improve Eye-Tracking
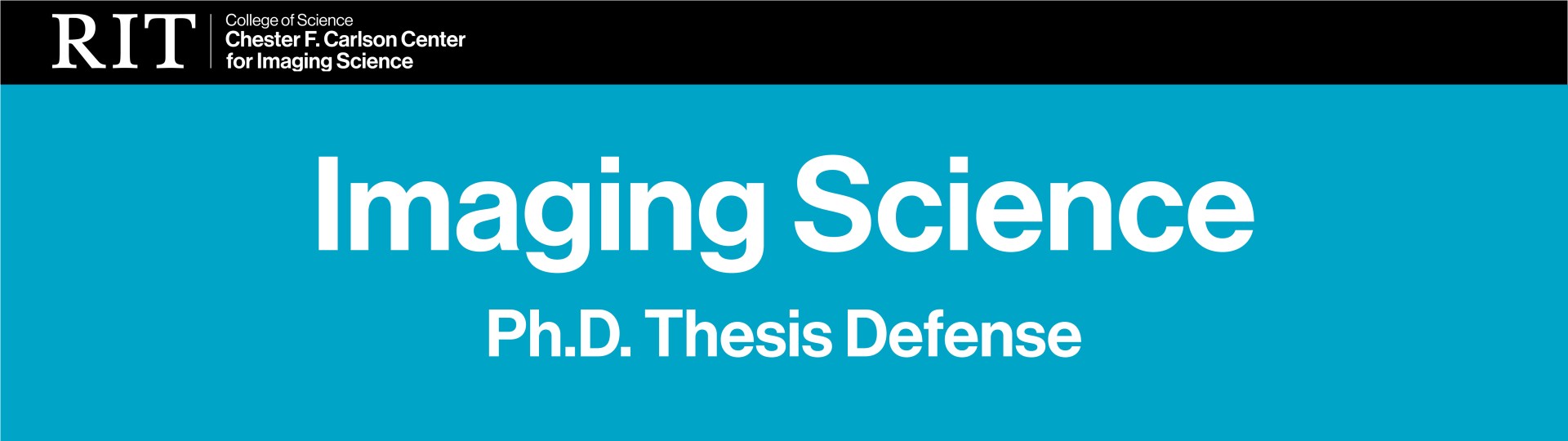
Ph.D. Dissertation Defense
Deep into the Eyes: Applying Machine Learning to improve Eye-Tracking
Aayush Kumar Chaudhary
Imaging Science Ph.D. Candidate
Chester F. Carlson Center for Imaging Science, RIT
Register Here for Zoom Link
This seminar may be attended in person in 2155 Carlson Building or online via Zoom.
Eye-tracking has been an active research area with applications in personal and behavioral studies, medical diagnosis, virtual reality, and mixed reality applications. In this dissertation, the speaker will demonstrate (I) a new method to improve precision while maintaining the accuracy of head-fixed eye trackers by combining velocity information from iris textures across frames with position information, (II) a generalized semantic segmentation framework for identifying eye regions with a further extension to identify ellipse fits on the pupil and iris, (III) a data-driven rendering pipeline to generate a temporally contiguous synthetic dataset for use in many eye-tracking applications, and (IV) a novel strategy to preserve privacy in eye videos captured as part of the eye-tracking process
Abstract:
Eye-tracking has been an active research area with applications in personal and behavioral studies, medical diagnosis, virtual reality, and mixed reality applications. Improving the robustness, generalizability, accuracy, and precision of eye-trackers while maintaining privacy is crucial. Unfortunately, many existing low-cost portable commercial eye trackers suffer from signal artifacts and a low signal-to-noise ratio. These trackers are highly dependent on low-level features such as pupil edges or diffused bright spots in order to precisely localize the pupil and corneal reflection. As a result, they are not reliable for studying eye movements that require high precision, such as microsaccades, smooth pursuit, and vergence. Additionally, these methods suffer from reflective artifacts, occlusion of the pupil boundary by the eyelid and often require a manual update of person-dependent parameters to identify the pupil region. In this dissertation, I demonstrate (I) a new method to improve precision while maintaining the accuracy of head-fixed eye trackers by combining velocity information from iris textures across frames with position information, (II) a generalized semantic segmentation framework for identifying eye regions with a further extension to identify ellipse fits on the pupil and iris, (III) a data-driven rendering pipeline to generate a temporally contiguous synthetic dataset for use in many eye-tracking applications, and (IV) a novel strategy to preserve privacy in eye videos captured as part of the eye-tracking process. My work also provides the foundation for future research by addressing critical questions like the suitability of using synthetic datasets to improve eye-tracking performance in real-world applications, and ways to improve the precision of future commercial eye trackers with improved camera specifications.
Intended Audience:
Undergraduates, graduates, and experts. Those with interest in the topic.
To request an interpreter, please visit https://myaccess.rit.edu
Event Snapshot
When and Where
Who
Open to the Public
Interpreter Requested?
No