Imaging Science Ph.D. Defense: Tyler Hayes
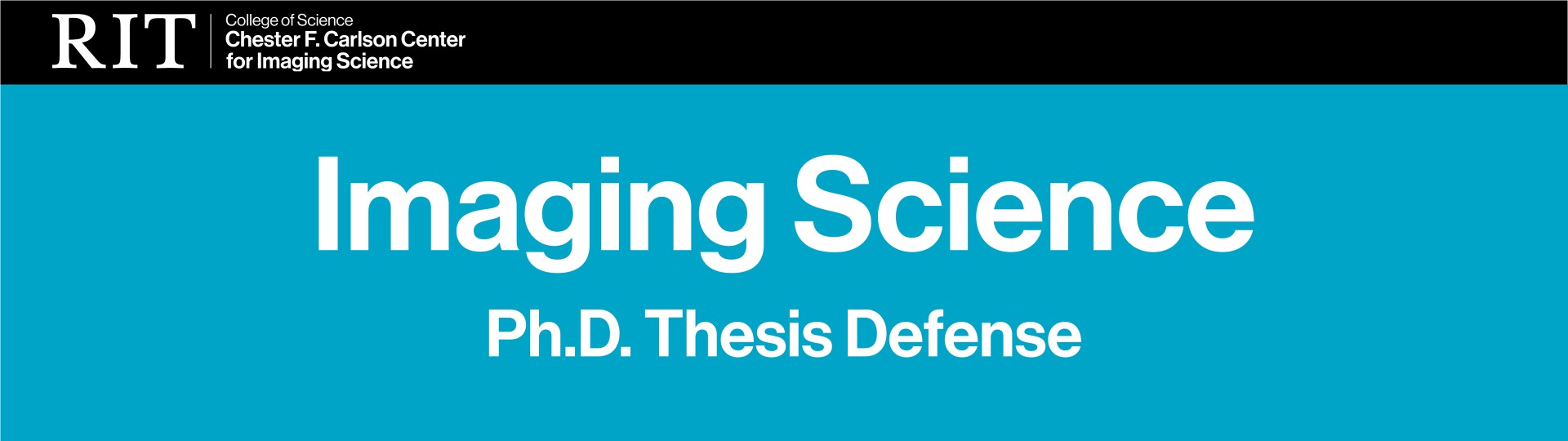
Ph.D. Dissertation Defense
Towards Efficient Lifelong Machine Learning in Deep Neural Networks
Tyler Hayes
Imaging Science Ph.D. Candidate
Chester F. Carlson Center for Imaging Science, RIT
Register Here for Zoom Link
This defense may be attended in-person in 3215 Carlson Building or online via Zoom.
By enabling Deep Neural Networks (DNNs) to learn new information over time, new adaptive applications for DNNs can be explored. These methods are applied to several visual and multi-modal perception tasks including image classification, visual question answering, analogical reasoning, and attribute and relationship prediction in visual scenes.
Abstract:
Humans continually learn and adapt to new knowledge and environments throughout their lifetimes. Rarely does learning new information cause humans to catastrophically forget previous knowledge. While deep neural networks (DNNs) now rival human performance on several supervised machine perception tasks, when updated on changing data distributions, they catastrophically forget previous knowledge. Enabling DNNs to learn new information over time opens the door for new applications such as self-driving cars that adapt to seasonal changes or smartphones that adapt to changing user preferences. In this dissertation, we propose new methods and experimental paradigms for efficiently training continual DNNs without forgetting. We then apply these methods to several visual and multi-modal perception tasks including image classification, visual question answering, analogical reasoning, and attribute and relationship prediction in visual scenes.
Intended Audience:
Undergraduates, graduates, and experts. Those with interest in the topic.
To request an interpreter, please visit https://myaccess.rit.edu
Event Snapshot
When and Where
Who
Open to the Public
Interpreter Requested?
No