Imaging Science MS Thesis Defense: Robert Chancia
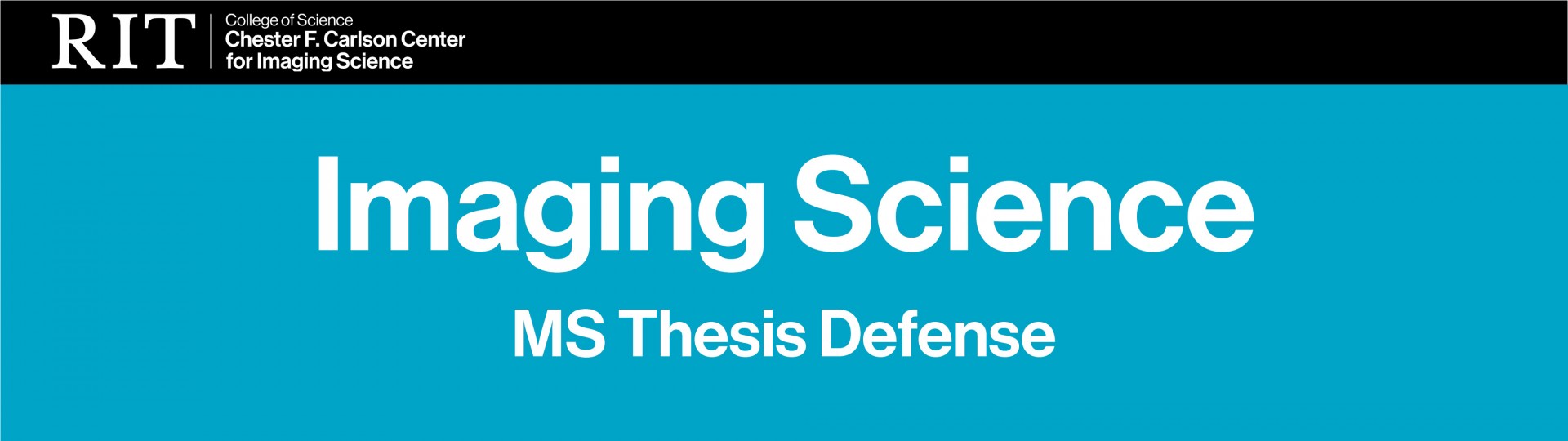
MS Thesis Defense
Improved crop nutrient and yield management using spectral sensing with unmanned aerial systems (UAS)
Robert Chancia
Imaging Science MS Candidate
Chester F. Carlson Center for Imaging Science, RIT
Abstract:
Remote sensing applications in agriculture are established for large-scale monitoring with satellite and airborne imagery but unmanned aerial systems (UAS) are poised to bring in-field mapping capabilities to individual farmers. UAS imaging boasts several advantages over traditional methods, including centimeter-scale resolution, reduced atmospheric absorption, flexible timing of data acquisitions, and ease of use. In this work, we present two studies using UAS imaging of specialty crops in upstate New York, with a focus on improving crop management practices. The first study involves root yield modeling, based on MicaSense 5-band multispectral imagery obtained by RIT over table beet fields in Batavia, NY, during the 2018 and 2019 seasons. We determined imagery obtained prior to canopy closing is ideal for yield predictions. Our root mass model based on area-augmented imagery of our raw study plots in 2018 had a root-mean-squared error of 0.6 kg/m2 and predicted a yield of 41,000 kg/ha on independent imagery from 2019 (typical yield for upstate New York is ~40,000 kg/ha). The second study was designed to determine an optimal subset of wavelengths related to grapevine nutrients for vineyard nutrient monitoring. RIT’s drone team flew both Headwall Nano and Micro Hyperspec imaging spectrometers over a Concord vineyard in Portland, NY in September of 2020, to obtain canopy spectra ranging from 400-2500 nm. Our ensemble wavelength selection algorithm selected wavelengths consistent with known absorption features related to nitrogen content in vegetation. Our regression model achieved a leave-one-out cross-validation root-mean-squared error of 0.17% for nitrogen in our dried vine-leaf samples, containing 2.4-3.6% nitrogen. This is an improvement upon published studies of typical UAS multispectral sensors used to assess grapevine nitrogen status. With further testing on new data, we can determine consistently selected wavelengths and guide the design of specialty multispectral sensors for improved grapevine nutrient management.
Intended Audience:
Undergraduates, graduates, and experts. Those with interest in the topic.
To request an interpreter, please visit https://myaccess.rit.edu
Event Snapshot
When and Where
Who
Open to the Public
Interpreter Requested?
No