Imaging Science Ph.D. Thesis Defense: Bei Cao
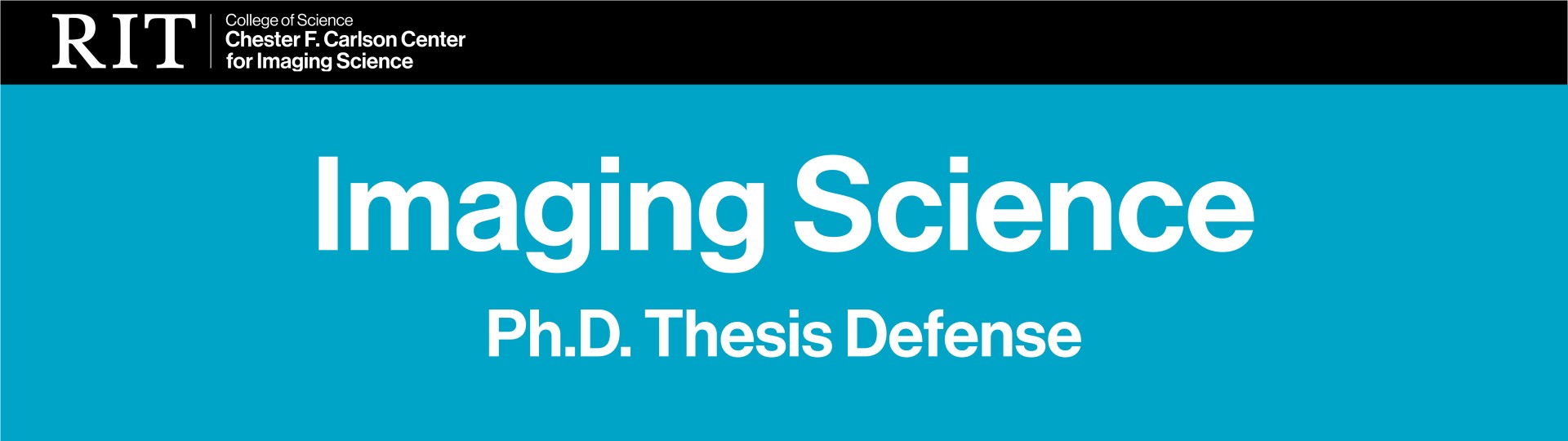
Ph.D. Dissertation Defense
Graph-based Learning Networks for Automatic Pigment Mapping of Historical Artifacts
Bei Cao
Imaging Science Ph.D. Candidate
Chester F. Carlson Center for Imaging Science, RIT
Abstract: Hyperspectral image classification can be a difficult task due to a lack of ground-truth labels and spectral variability. For hyperspectral images (HSIs) of historical artifacts, which are generally man-made objects, this is generally a significant challenge. Aiming to differentiate subtle spectral differences between varied pigments, we introduce graph-based learning networks and a powerful learning pipeline for realizing automatic pigment mapping on the maps. First, we develop a Graph Modularity clustering method for producing trustworthy ground-truth map for pigment classification task. We analyze the influence of graph structure on the Graph Neural Networks and propose Spatial-Spectral Graph Convolutional Network (SSGCN) and Spatial-Spectral Graph Attention Network (SSGAT). By incorporating spatial features and aggregating node feature in terms of spectral affinity and spatial context, SSGCN and SSGAT outperform conventional GCN and GAT, and can be directly applied to another unknown region for automatic pigment mapping without ground-truth labels required. Under a semi-supervised node classification framework, SSGCN and SSGAT achieve high classification accuracy with very limited labeled samples. Compared to a 3D-CNN architecture, our graph-based learning models provide great scalability in model extension and inductive learning for unseen pixels. We apply these methods to Vis-NIR hyperspectral imagery of the Gough Map of Great Britain and the Selden Map of China, both artifacts in the Bodleian Library, University of Oxford. These maps are of great interest to historians as their creation and modification are generally not well understood. The experiments on multiple Regions of Interest in the two maps demonstrate the strength and advantages of our methods. The proposed graph-based learning frameworks bring a promising prospect for processing large-scale HSI classification/clustering of historical artifacts efficiently. This research will aid historians in pigment analysis and codicological studies of artifacts in a more intelligent manner.
Intended Audience: Undergraduates, graduates, and experts. Those with interest in the topic.
To request an interpreter, please visit https://myaccess.rit.edu
Event Snapshot
When and Where
Who
Open to the Public
Interpreter Requested?
No