Inverse Problems Seminar: Deterministic and Probabilistic Estimation of Cardiac Electrophysiological Model Parameters via Bayesian Active Learning
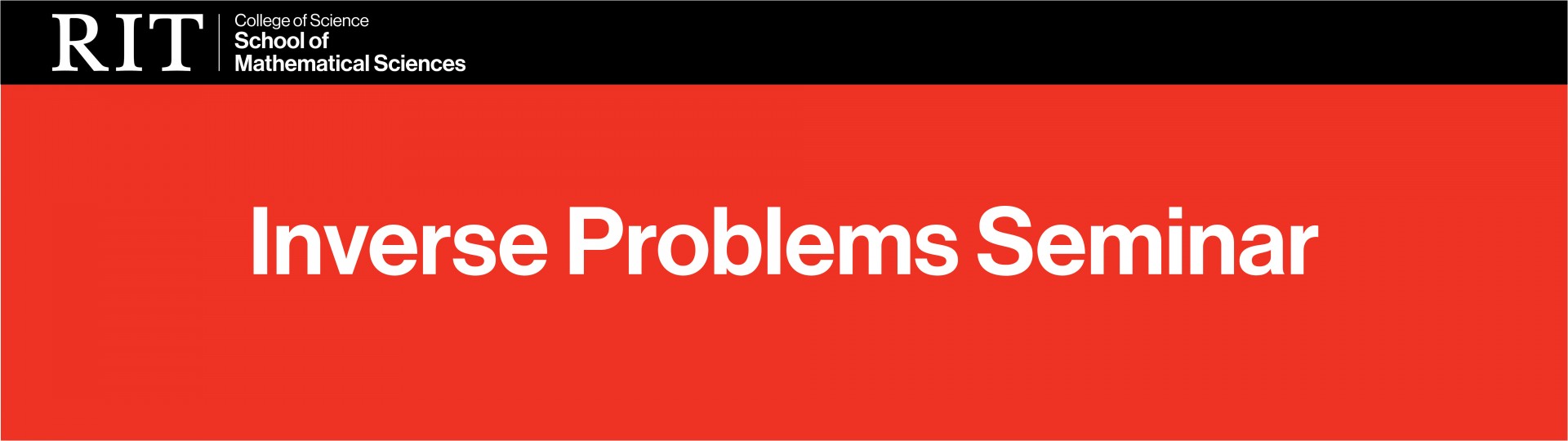
Inverse Problems Seminar
Deterministic and Probabilistic Estimation of Cardiac Electrophysiological Model Parameters via Bayesian Active Learning
Md Shakil Zaman & Pradeep Bajracharya
Ph. D. Candidates
Computing & Information Science Ph. D. Program, RIT
Register Here for Zoom Link
Abstract:
The deterministic and probabilistic estimation of patient-specific tissue properties in the form of model parameters is important for personalized physiological models. The expensive computation associated with these model simulations, however, makes direct Markov Chain Monte Carlo (MCMC) sampling inefficient for both deterministic optimization and probabilistic estimation of model parameters. Approximated models resulting from replacing the simulation model with a computationally efficient surrogate, on the other hand, have seen limited accuracy. In this work, we present a Bayesian active learning method to directly approximate (1) the mode as deterministic optimization and (2) the posterior probability density function (pdf) as probabilistic estimation of cardiac model parameters with small number of samples where we intelligently select training points to query the expensive simulation process. We integrate a generative model into Bayesian active learning to allow high-dimensional model parameter estimation in low-dimensional space. Furthermore we introduce new acquisition functions to focus the selection of training points in this Bayesian active learning. We evaluated the presented methods in estimating tissue excitability in a 3D cardiac electrophysiological model in a range of synthetic and real-data experiments. Our method demonstrated its improved accuracy in approximating the mode and the posterior pdf compared to Bayesian active learning using regular acquisition functions, and substantially reduced computational cost in comparison to existing standard or accelerated MCMC sampling.
Intended Audience:
Undergraduates, graduates, and experts. Those with interest in the topic.
Keep up with Inverse Problems Seminars on the Inverse Problems Seminar webpage.
To request an interpreter, please visit myaccess.rit.edu
Event Snapshot
When and Where
Who
This is an RIT Only Event
Interpreter Requested?
No