Ph.D. Thesis Defense: Austin Bergstrom
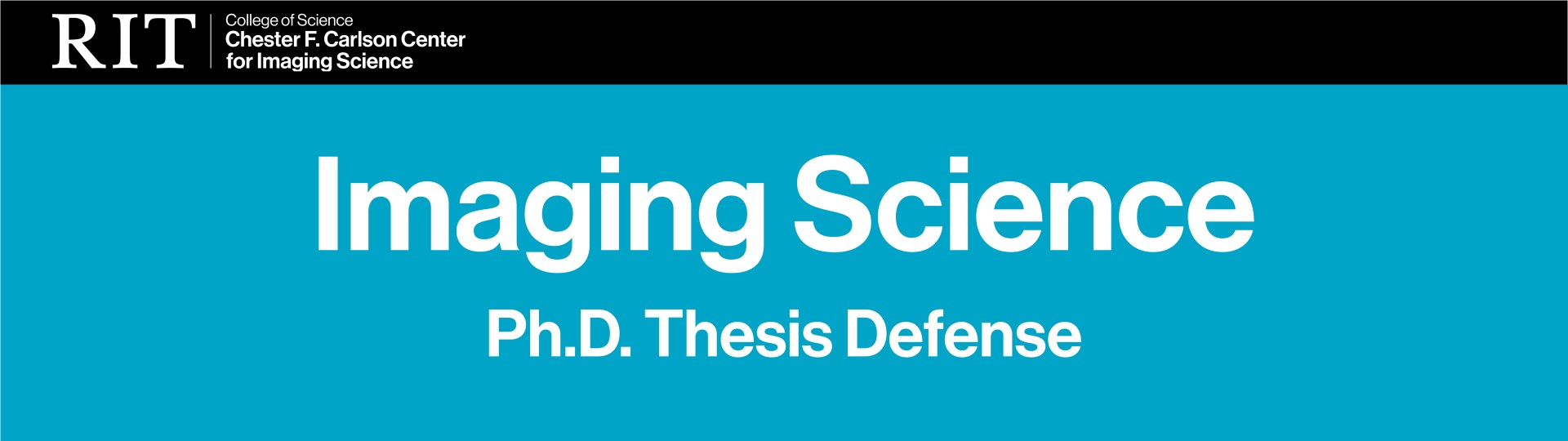
Ph.D. Thesis Defense
Understanding the Relationship Between Image Quality and Computer Vision Performance
Austin Bergstrom
Imaging Science PhD Candidate
Chester F. Carlson Center for Imaging Science, RIT
Abstract: Extensive research has gone into optimizing convolutional neural network (CNN) architectures for tasks such as image classification and object detection, but research to date on the relationship between input image quality and CNN prediction performance has been relatively limited. Additionally, studies on the topic to date suggest that the relationship between image quality and CNN performance differs from the relationship between image quality and ease of human interpretation. Furthermore, CNN generalization against out-of-distribution image distortions persists as a significant challenge and a focus of substantial research, but a range of studies suggest that CNNs can be be made robust to low visual quality images when the distortions are predictable. This research examines the affects of image resolution, blur, and noise on CNN performance in classification and object detection tasks. We find that while generalization remains a significant challenge for CNNs faced with out-of-distribution image distortions, CNN performance against low visual quality images remains strong with appropriate training, indicating the potential to expand the design trade space for sensors providing data to computer vision systems. We find that the functional form of the GIQE can predict CNN performance as a function of image degradation, but we observe that the legacy form of the GIQE does a better job of modeling the impact of blur/relative edge response in some scenarios. Additionally, we evaluate other image quality models that lack the pedigree of the GIQE and find that they generally work as well or better than the functional form of the GIQE in modeling computer vision performance on distorted images. We observe qualitatively similar distortion performance relationships across image classifiers and object detectors.
Intended Audience: Undergraduates, graduates, and experts. Those with interest in the topic.
Event Snapshot
When and Where
Who
Open to the Public
Interpreter Requested?
No