Data Science Master of Science Degree
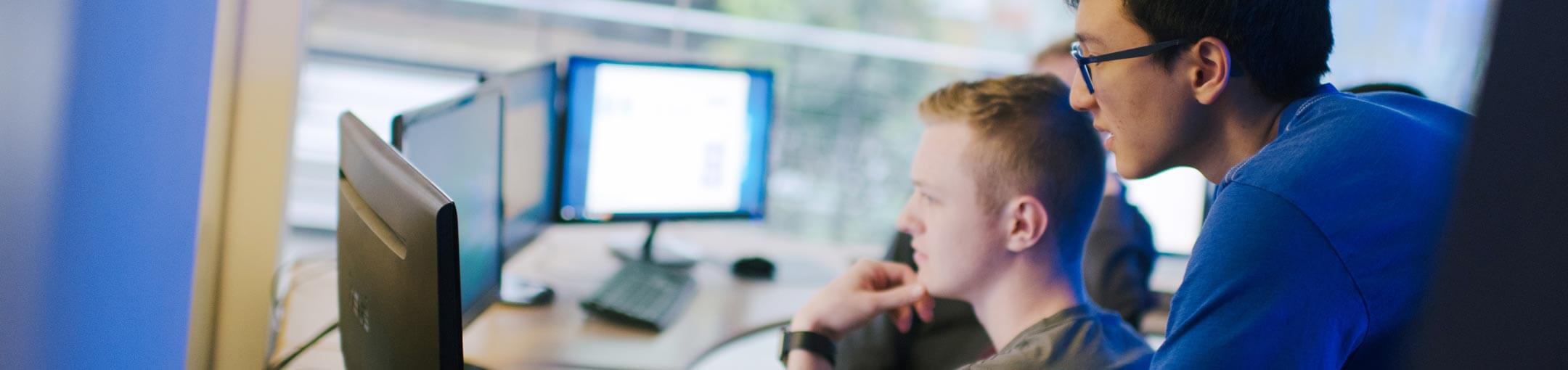
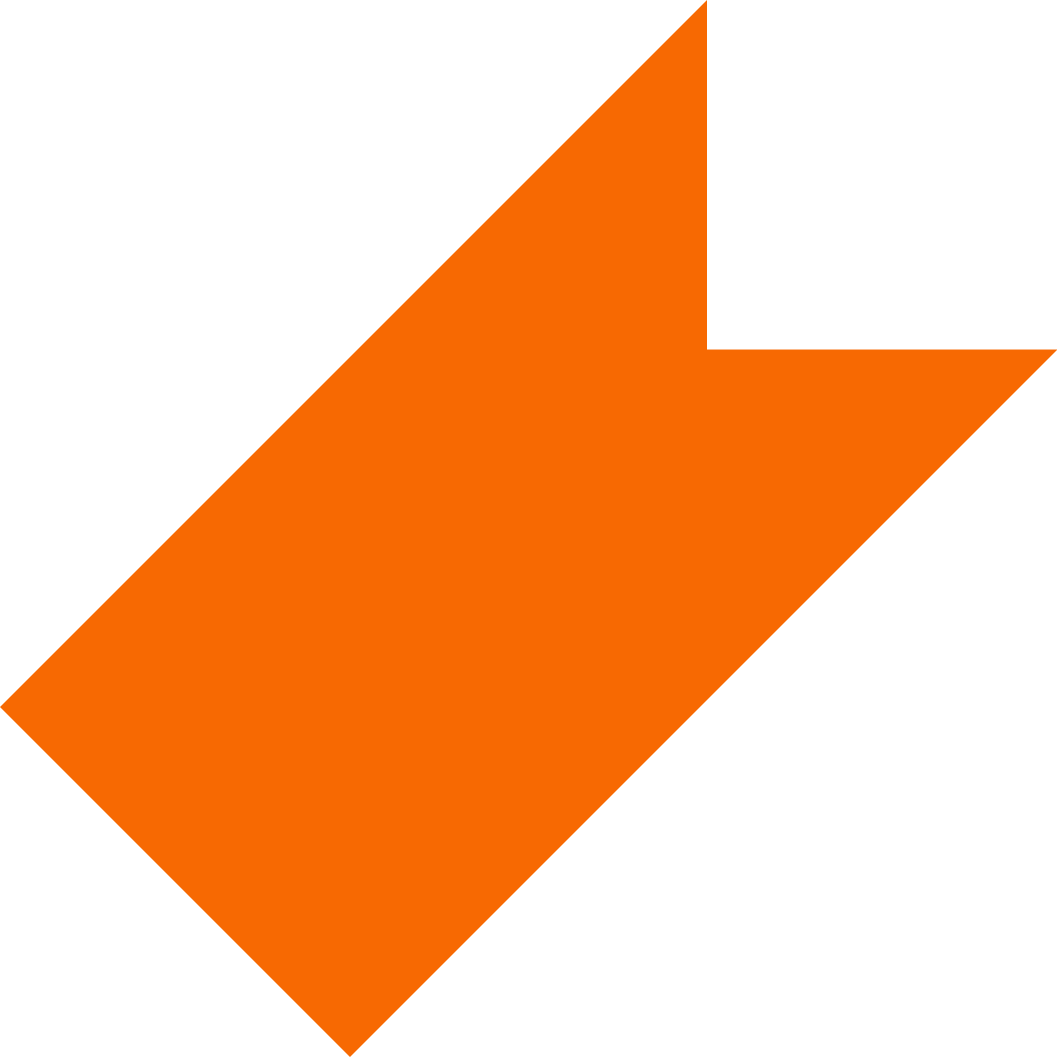
Data Science
Master of Science Degree
- RIT /
- Rochester Institute of Technology /
- Academics /
- Data Science MS
Request Info about graduate study
Visit
Apply
Travis Desell
Erik Golen
Demand is high for professionals skilled in both analytics and computing. Enhance your skill set by learning to manage large-scale data sets in this data science master’s.
Overview for Data Science MS
Data science is one of the hottest fields in computing. The data science degree gives you the practical and theoretical skills to handle large-scale data management and analysis challenges that arise in today’s data-driven organizations. This program appeals to professionals looking to enhance their skill set, and includes opportunities for customized course work within the broad field of data science and its various application areas.
RIT’s Data Science Master’s: On-Campus or Online
In response to the growing need to generate and analyze meaningful data across all industries, demand is on the rise for a new breed of professionals skilled in both analytics and computing. RIT’s MS in data science encourages you to work with faculty experts in the fields of data science, analytics, and infrastructure who provide hands-on experience solving real problems. The curriculum includes opportunities for you to choose elective courses to pursue a variety of career paths within the broad field of data science and its various application areas. The program prepares you—regardless of your scientific, engineering, or business background—to pursue a career in data science.
RIT’s colleges of Science and Computing and Information Sciences collaborated to deliver the data science master's, which combines the expertise and knowledge from faculty in both colleges to provide you with a unique understanding of math, computing, and technology. This approach enhances your learning outcomes and increases career marketability.
What You'll Learn
- Concepts and skills in machine learning to prepare you to build, tune, and discover actionable insights from predictive models
- Programming language skills in Python and Java to be able to synthesize large unstructured data sets
- Competencies in data mining, regression analysis, text mining, and predictive analytics
- How to create, critically assess, interpret, and communicate rich visualizations
A Data Science Master’s Curriculum Packed with High-Demand Skills
The explosive growth in demand for data science skills is disrupting today’s job markets. These skills are found in over half of all job postings related to this field.
- Data Analytics: Data analysis skills are projected to grow in demand to 82% by 2026, and machine learning skills are growing by 1-2%.
- Data Science: Demand for skills in artificial intelligence is growing by 128% and deep learning skills demand is growing by 135%.
- Software and Programming: Demand for expertise in Python is growing by 21%; blockchain skills are growing by 245%.
- Experimental Design: Crossover, adaptive, and equivalence designs are dominating 38% of this job market.
Designed for working professionals studying on campus or online part-time, this degree program has a strong career focus. You’ll learn both practical and theoretical skills to handle large-scale data management and analysis challenges ever-present in today’s data-driven organizations. This program places a unique focus on training data scientists in strong software engineering skills so that they can effectively develop real-world data science applications which operate within modern organizations' computational workflows. You’ll be learning with students from varied professional backgrounds and working with practitioners active in the field to provide hands-on experience solving real problems.
Students are also interested in: Information Technology and Analytics MS, Business Analytics MS, Applied Statistics MS, Health Informatics MS, Bioinformatics MS
Join us for Fall 2024
Many programs accept applications on a rolling, space-available basis.
30% Tuition Scholarship for NY Residents and Graduates
Now is the perfect time to earn your Master’s degree. If you’re a New York state resident with a bachelor’s degree or have/will graduate from a college or university in New York state, you are eligible to receive a 30% tuition scholarship.
Careers and Cooperative Education
Typical Job Titles
Data Scientist | Data Engineer |
Data Architect | Machine Learning Engineer |
Data Analyst | Database Administrator |
Statistician | Business Analyst |
Cooperative Education
What makes an RIT education exceptional? It’s the ability to complete relevant, hands-on career experience. At the graduate level, and paired with an advanced degree, cooperative education and internships give you the unparalleled credentials that truly set you apart. Learn more about graduate co-op and how it provides you with the career experience employers look for in their next top hires.
Cooperative education is optional but strongly encouraged for graduate students in the data science MS degree.
Featured Work
Student researchers pave the way for human-centered AI advances in the College of Liberal Arts Computational Linguistics and Speech Processing Lab.
Student summer researchers work to build a mobile app for interactive machine learning and visualization tool to verify data in support of human-centered models
Featured Profiles
Your Partners in Success: Meet Our Faculty, Dr. Wong
Dr. Tony Wong
Mathematics is a powerful tool for answering questions. From mitigating climate risks to splitting the dinner bill, Professor Wong shows students that math is more than just a prerequisite.
Curriculum for 2023-2024 for Data Science MS
Current Students: See Curriculum Requirements
Data Science, MS degree, typical course sequence (on-campus program)
Course | Sem. Cr. Hrs. | |
---|---|---|
First Year | ||
DSCI-601 | Applied Data Science I This is the first of a two course applied data science seminar series. Students will be introduced to the data science masters program along with potential projects which they will develop over the course of this series in con-junction with the applied data science directed studies. Students will select a project along with an advisor and sponsor, develop a written proposal for their work, and investigate and write a related work survey to refine this proposal with their findings. Students will begin preliminary design and implementation of their project. Work will be presented in class for peer review with an emphasis on developing data science communication skills. This course will keep students up to date with the broad range of data science applications. (Prerequisites: SWEN-601 and DSCI-633 and STAT-614 or equivalent courses.) Lecture 3 (Fall). |
3 |
DSCI-633 | Foundations of Data Science and Analytics A foundations course in data science, emphasizing both concepts and techniques. The course provides an overview of data analysis tasks and the associated challenges, spanning data preprocessing, model building, model evaluation, and visualization. The major areas of machine learning, such as unsupervised, semi-supervised and supervised learning are covered by data analysis techniques including classification, clustering, association analysis, anomaly detection, and statistical testing. The course includes a series of assignments utilizing practical datasets from diverse application domains, which are designed to reinforce the concepts and techniques covered in lectures. A substantial project related to one or more data sets culminates the course. (This course is restricted to DATASCI-MS, INFOST-MS, SOFTENG-MS, COMPSCI-MS, or COMPIS-PHD Major students.) Lecture 3 (Fall, Spring). |
3 |
DSCI-644 | Software Engineering for Data Science This course focuses on the software engineering challenges of building scalable and highly available big data software systems. Software design and development methodologies and available technologies addressing the major software aspects of a big data system including software architectures, application design patterns, different types of data models and data management, and deployment architectures will be covered in this course. (Prerequisites: SWEN-601 and DSCI-633 or equivalent courses.) Lecture 3 (Spring). |
3 |
ISTE-608 | Database Design And Implementation An introduction to the theory and practice of designing and implementing database systems. Current software environments are used to explore effective database design and implementation concepts and strategies. Topics include conceptual data modeling, methodologies, logical/physical database design, normalization, relational algebra, schema creation and data manipulation, and transaction design. Database design and implementation projects are required. Lec/Lab 4 (Fall, Spring). |
3 |
STAT-614 | Applied Statistics Statistical tools for modern data analysis can be used across a range of industries to help you guide organizational, societal and scientific advances. This course is designed to provide an introduction to the tools and techniques to accomplish this. Topics covered will include continuous and discrete distributions, descriptive statistics, hypothesis testing, power, estimation, confidence intervals, regression, one-way ANOVA and Chi-square tests. (This class is restricted to degree-seeking graduate students or those with permission from instructor.) Lecture 3 (Fall). |
3 |
SWEN-601 | Software Construction This is a programming based course to enhance individual, technical engineering knowledge and skills as preparation for masters level graduate work in computing. Students will be introduced to programming language syntax, object oriented concepts, data structures and foundational algorithms. An emphasis will be placed on obtaining practical programming skills, through regular programming assignments and practicum. (Corequisites: SWEN-610 and SWEN-746 or equivalent courses.) Lecture 3 (Fall). |
3 |
Electives |
3 | |
Second Year | ||
DSCI-602 | Applied Data Science II This is the second of a three course applied data science seminar series. Students will design an implementation plan and preliminary documentation for their selected applied data science project, along with an in class presentation of this work. At the end of the semester students will present preliminary demos of their project and write a preliminary project report. Writing and presentations will be peer reviewed to further enhance data science communication skills. This course will keep students up to date with the broad range of data science applications. (Prerequisite: DSCI-601 or equivalent course.) Lecture 3 (Spring). |
3 |
Electives |
6 | |
Total Semester Credit Hours | 30 |
Data Science, MS degree, typical course sequence (online program)
Course | Sem. Cr. Hrs. | |
---|---|---|
First Year | ||
DSCI-633 | Foundations of Data Science and Analytics A foundations course in data science, emphasizing both concepts and techniques. The course provides an overview of data analysis tasks and the associated challenges, spanning data preprocessing, model building, model evaluation, and visualization. The major areas of machine learning, such as unsupervised, semi-supervised and supervised learning are covered by data analysis techniques including classification, clustering, association analysis, anomaly detection, and statistical testing. The course includes a series of assignments utilizing practical datasets from diverse application domains, which are designed to reinforce the concepts and techniques covered in lectures. A substantial project related to one or more data sets culminates the course. (This course is restricted to DATASCI-MS, INFOST-MS, SOFTENG-MS, COMPSCI-MS, or COMPIS-PHD Major students.) Lecture 3 (Fall, Spring). |
3 |
ISTE-608 | Database And Implementation An introduction to the theory and practice of designing and implementing database systems. Current software environments are used to explore effective database design and implementation concepts and strategies. Topics include conceptual data modeling, methodologies, logical/physical database design, normalization, relational algebra, schema creation and data manipulation, and transaction design. Database design and implementation projects are required. Lec/Lab 4 (Fall, Spring). |
3 |
STAT-614 | Applied Statistics Statistical tools for modern data analysis can be used across a range of industries to help you guide organizational, societal and scientific advances. This course is designed to provide an introduction to the tools and techniques to accomplish this. Topics covered will include continuous and discrete distributions, descriptive statistics, hypothesis testing, power, estimation, confidence intervals, regression, one-way ANOVA and Chi-square tests. (This class is restricted to degree-seeking graduate students or those with permission from instructor.) Lecture 3 (Fall). |
3 |
SWEN-601 | Software Construction This is a programming based course to enhance individual, technical engineering knowledge and skills as preparation for masters level graduate work in computing. Students will be introduced to programming language syntax, object oriented concepts, data structures and foundational algorithms. An emphasis will be placed on obtaining practical programming skills, through regular programming assignments and practicum. (Corequisites: SWEN-610 and SWEN-746 or equivalent courses.) Lecture 3 (Fall). |
3 |
Elective |
3 | |
Second Year | ||
DSCI-644 | Software Engineering for Data Science This course focuses on the software engineering challenges of building scalable and highly available big data software systems. Software design and development methodologies and available technologies addressing the major software aspects of a big data system including software architectures, application design patterns, different types of data models and data management, and deployment architectures will be covered in this course. (Prerequisites: SWEN-601 and DSCI-633 or equivalent courses.) Lecture 3 (Spring). |
3 |
DSCI-799 | Graduate Capstone This non-class-based experience provides the student with an individual opportunity to explore a project-based or a research-based project that advances knowledge in an area of data science. The student selects a problem, conducts background research, develops the system or devises a research approach, analyses the results, and builds a professional document and presentation that disseminates the project. The report must include a literature review. The final report structure is to be determined by the capstone advisor. Ind Study (Fall, Spring, Summer). |
3 |
Electives |
9 | |
Total Semester Credit Hours | 30 |
Data Science, MS degree, typical course sequence (online + edX program)
Course | Sem. Cr. Hrs. | |
---|---|---|
First Year | ||
ISTE-608 | Database Design And Implementation An introduction to the theory and practice of designing and implementing database systems. Current software environments are used to explore effective database design and implementation concepts and strategies. Topics include conceptual data modeling, methodologies, logical/physical database design, normalization, relational algebra, schema creation and data manipulation, and transaction design. Database design and implementation projects are required. Lec/Lab 4 (Fall, Spring). |
3 |
SWEN-601 | Software Construction This is a programming based course to enhance individual, technical engineering knowledge and skills as preparation for masters level graduate work in computing. Students will be introduced to programming language syntax, object oriented concepts, data structures and foundational algorithms. An emphasis will be placed on obtaining practical programming skills, through regular programming assignments and practicum. (Corequisites: SWEN-610 and SWEN-746 or equivalent courses.) Lecture 3 (Fall). |
3 |
edX Micromasters |
9 | |
Elective |
3 | |
Second Year | ||
DSCI-644 | Software Engineering for Data Science This course focuses on the software engineering challenges of building scalable and highly available big data software systems. Software design and development methodologies and available technologies addressing the major software aspects of a big data system including software architectures, application design patterns, different types of data models and data management, and deployment architectures will be covered in this course. (Prerequisites: SWEN-601 and DSCI-633 or equivalent courses.) Lecture 3 (Spring). |
3 |
DSCI-799 | Graduate Capstone This non-class-based experience provides the student with an individual opportunity to explore a project-based or a research-based project that advances knowledge in an area of data science. The student selects a problem, conducts background research, develops the system or devises a research approach, analyses the results, and builds a professional document and presentation that disseminates the project. The report must include a literature review. The final report structure is to be determined by the capstone advisor. Ind Study (Fall, Spring, Summer). |
3 |
Electives |
6 | |
Total Semester Credit Hours | 30 |
Note for online students
The frequency of required and elective course offerings in the online program will vary, semester by semester, and will not always match the information presented here. Online students are advised to seek guidance from the listed program contact when developing their individual program course schedule.
Admissions and Financial Aid
This program is available on-campus or online.
On Campus
Offered | Admit Term(s) | Application Deadline | STEM Designated |
---|---|---|---|
Full-time | Fall | Rolling | Yes |
Part-time | Fall | Rolling | No |
Online
Offered | Admit Term(s) | Application Deadline | STEM Designated |
---|---|---|---|
Part-time | Fall or Spring | Rolling | No |
Full-time study is 9+ semester credit hours. Part-time study is 1‑8 semester credit hours. International students requiring a visa to study at the RIT Rochester campus must study full‑time.
Application Details
To be considered for admission to the Data Science MS program, candidates must fulfill the following requirements:
- Complete an online graduate application.
- Submit copies of official transcript(s) (in English) of all previously completed undergraduate and graduate course work, including any transfer credit earned.
- Hold a baccalaureate degree (or US equivalent) from an accredited university or college.
- A recommended minimum cumulative GPA of 3.0 (or equivalent).
- Submit a current resume or curriculum vitae.
- Submit a personal statement of educational objectives.
- Submit two letters of recommendation.
- Entrance exam requirements: GRE required for individuals with degrees from international universities. No minimum score requirement.
- Writing samples are optional.
- Submit English language test scores (TOEFL, IELTS, PTE Academic), if required. Details are below.
English Language Test Scores
International applicants whose native language is not English must submit one of the following official English language test scores. Some international applicants may be considered for an English test requirement waiver.
TOEFL | IELTS | PTE Academic |
---|---|---|
88 | 6.5 | 60 |
International students below the minimum requirement may be considered for conditional admission. Each program requires balanced sub-scores when determining an applicant’s need for additional English language courses.
How to Apply Start or Manage Your Application
Cost and Financial Aid
An RIT graduate degree is an investment with lifelong returns. Graduate tuition varies by degree, the number of credits taken per semester, and delivery method. View the general cost of attendance or estimate the cost of your graduate degree.
A combination of sources can help fund your graduate degree. Learn how to fund your degree
Additional Information
Prerequisites
It is expected that prospective students will have a sufficient background in object-oriented programming. However, accepted students without this prerequisite knowledge may complete prescribed bridge course(s) that are available.
Bridge Courses
Students whose undergraduate preparation or employment experience does not satisfy the prerequisites can make up for this by completing prerequisite bridge course(s), as prescribed by the graduate program director. The bridge course(s) are not part of the 30-semester credit hours required for the master’s degree. Grades for bridge course(s) are not included in a student’s GPA if taken before matriculation; they are included if completed after matriculation. Since bridge programs can be designed in a variety of ways, the graduate program director will assist students in planning and course selection.
Online Degree Information
The online Data Science MS program can only be completed part-time, taking one or two courses per term. The average time to completion is two and a half to three years. For the fully online Data Science MS, core classes are offered asynchronously, as are many electives. Students can complete the degree without any synchronous courses. Program electives are slightly more limited than courses available in the on-campus program, and some may be synchronous only. Any live group discussions or class meetings are typically optional and may be recorded, and students should connect with their professor to obtain the material. Students typically spend 10-12 hours per week per class, depending on the content and their background knowledge. The online program culminates with a capstone course and capstone project. For specific details about the delivery format and learning experience, contact the Program Contact listed on this page. RIT does not offer student visas for online study.
Online Tuition Eligibility
The online Data Science MS is a designated online degree program that is billed at a 43% discount from our on-campus rate. View the current online tuition rate.
Online Study Restrictions for Some International Students
Certain countries are subject to comprehensive embargoes under US Export Controls, which prohibit virtually ALL exports, imports, and other transactions without a license or other US Government authorization. Learners from the Crimea region of the Ukraine, Cuba, Iran, North Korea, and Syria may not register for RIT online courses. Nor may individuals on the United States Treasury Department’s list of Specially Designated Nationals or the United States Commerce Department’s table of Deny Orders. By registering for RIT online courses, you represent and warrant that you are not located in, under the control of, or a national or resident of any such country or on any such list.
Latest News
-
April 29, 2024
Students discover research opportunities on the path to graduation
Independent research projects can help cultivate critical thinking, collaboration, and problem-solving skills. Whether it’s late nights spent in a RIT lab or a field study in the mountains, research experiences can be a cutting-edge way for students to prepare for the future.
-
April 3, 2023
RIT Master Plan gives graduate tuition scholarship to eligible alumni
RIT is offering a graduate tuition scholarship to recent alumni seeking to expand their career potential through the university’s graduate programs. Alumni can enhance their skill set for the new economy through master’s degrees that build upon collaboration, analytical thinking, complex problem solving, and flexibility.
-
January 9, 2023
Staying busy between semesters
During the 15 weeks between spring and fall semester, RIT students are finding ways to embrace new challenges. Some are taking the stage and performing. Others are winning club championships. For many, summer is a time to get work experience and participate in research projects, traveling abroad, and helping others while pursuing their passions.